Program Report: Labor Studies, 2020
The Labor Studies Program is one of the largest and most active in the NBER. Its nearly 190 members produce more than 300 working papers in an average year. The breadth and depth of questions addressed by Labor Studies members is immense. Research touches on macroeconomic topics such as unemployment and productivity; institutional factors such as minimum wage regulations, labor unions, and globalization; and technological developments including robotics, artificial intelligence, and algorithmic decision-making. It also includes core human capital subjects such as educational investment, the demand and supply of skills, and wage determination; industrial organization topics such as imperfect competition, rent sharing, and firm-specific wage policies; and social insurance and welfare programs such as unemployment insurance, universal basic income, and in-kind benefit programs such as SNAP, Medicaid, and housing assistance. Program affiliates also study urgent social questions, including race and gender disparities in market opportunities, neighborhood quality, treatment by the criminal justice system, and many other subject domains.
Reflecting their intellectual diversity, two-thirds of Labor Studies Program members are affiliated with two or more NBER programs or major projects. Though the pandemic has curtailed some program activities, it has simultaneously opened new horizons. The online meeting environment has allowed many nonaffiliated scholars to participate in program meetings. Meanwhile, researchers who prefer to audit rather than participate in program sessions can watch meetings streamed live on NBER’s YouTube channel. In the post-pandemic world, the program will strive to keep these professional and intellectual doors open.
This brief report summarizes a small subset of topics where research by Labor Studies affiliates is burgeoning, including the role of firms in wage determination; the minimum wage; the consequences of advancing technologies for employment and productivity; race and ethnicity in the labor market; and the extent and consequences of racial and ethnic discrimination and segregation. This summary does not do justice to the vast body of recent scholarship by program affiliates, though our hope is that it reveals some important research undercurrents.
Automation, Employment, and Productivity
The role of automation in shaping labor demand, skill requirements, and wage levels has been of intense economic interest for centuries. Even so, this topic has gained further prominence as rapid advances in ubiquitous computing, artificial intelligence, and robotics have imbued machines with the ability to accomplish tasks that require learning, judgment, and dexterity. Labor Studies scholars have taken numerous angles of attack to assess what this has meant for labor markets and to forecast what may lie ahead.
One influential paper in this domain by Daron Acemoglu and Pascual Restrepo explores how the expansion of industrial robotics has affected employment and wages in local labor markets — so-called commuting zones.1 Harnessing data on industrial robot penetration in other industrialized countries to measure the technological frontier, the researchers calculate predicted robot adoption in the United States within local labor markets based on initial industry structures in those locations. A key finding is that local labor markets with greater exposure to robot adoption saw differential falls in employment-to-population rates (and wages, not pictured) in the 1990s and early 2000s. An independent empirical contribution by George Borjas and Richard Freeman reaches a similar conclusion.2
Brad Hershbein and Lisa B. Kahn explore how recessions may accelerate the process of technological change by studying the evolution of skill requirements posted in job vacancies, using a vast database of vacancy postings scraped from the web by Burning Glass Technologies.3 They show that skill requirements in job vacancy postings differentially increased in metropolitan statistical areas that were hit hardest by the Great Recession, and these increases persisted through at least the end of 2015, long after the recession was over. They interpret this evidence as consistent with adjustment cost models in which adverse shocks accelerate the process of adaptation to new business processes, in this case, so-called routine-task-replacing technologies and the more-skilled workers who complement them. Consonant with these findings, Alex W. Chernoff and Casey Warman argue that the current COVID-19 pandemic may speed the process of automation. They further present evidence that in a large set of countries, the occupations held disproportionately by women are at greater risk of displacement by automation, implying that the post-pandemic labor market may offer fewer of the positions frequently held by women.4
Illuminating another facet of the interplay among technological change, demand shifts, and labor market adjustment, Elizabeth U. Cascio and Ayushi Narayan study the impact of the introduction of hydraulic fracturing (fracking) for oil extraction, a technology introduced during the 2000s, on educational investments.5 Because fracking offers high-paying blue-collar jobs to workers without secondary credentials, it potentially raises the opportunity cost of schooling. As theory would predict — and as many parents would lament — high school dropout rates rose among male teenagers living near shale oil deposits.
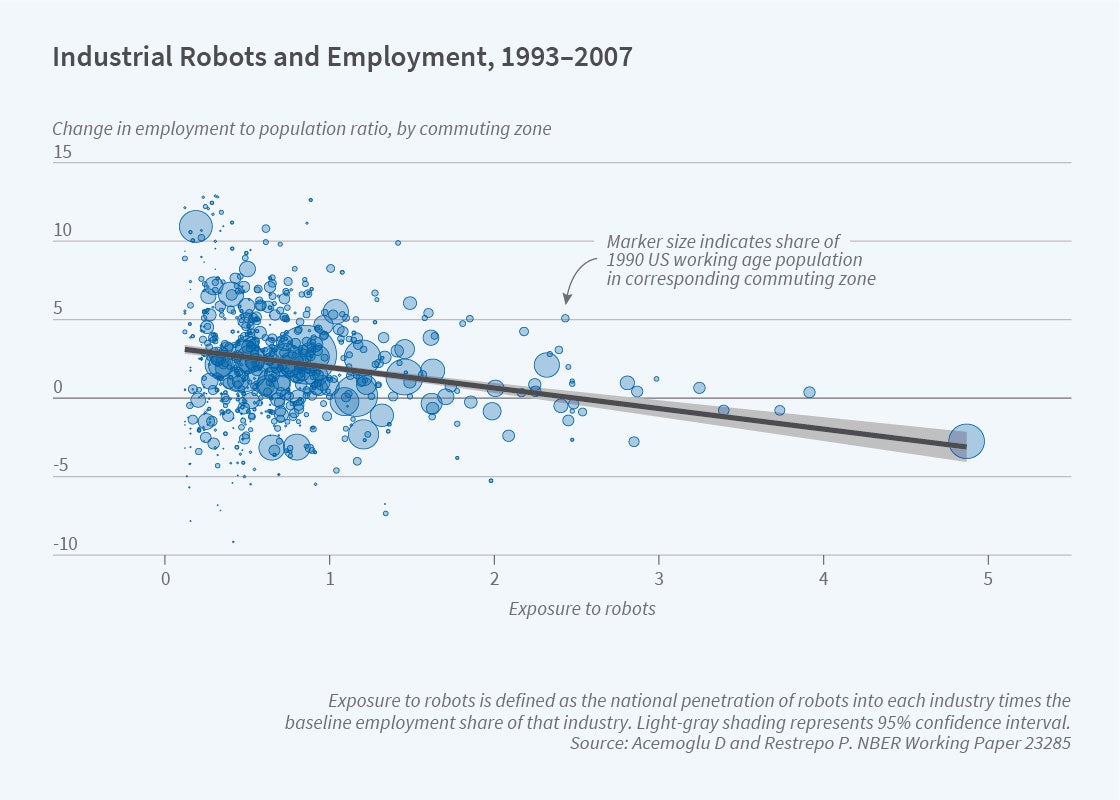
What are the long-run implications of advancing automation for skill demands? A theoretical paper by Seth G. Benzell, Laurence J. Kotlikoff, Guillermo LaGarda, and Jeffrey D. Sachs considers how, in an overlapping generation setting, automation can ultimately lead to worker immiseration by reducing capital formation as long-lived, barely depreciating software capital effectively makes high-skill workers redundant.6 In related work, Anton Korinek and Joseph E. Stiglitz consider the challenges that artificial intelligence may ultimately pose for income distribution and unemployment.7 David E. Bloom, Mathew McKenna, and Klaus Prettner place this issue in global perspective by observing that the global labor market will need to absorb roughly three-quarters of a billion new workers between 2010 and 2030.8 With 91 percent of that growth occurring in low- and lower-middle-income countries, they raise the concern that technological advances may create headwinds because the labor-intensive jobs currently prevalent in developing countries may be increasingly subject to automation.
While most of the papers above focus on the economic implications of machines substituting for labor, work by David Deming presents evidence that as automation proceeds, the demand for human capabilities is rising on another margin: social and managerial skills.9 Deming argues that as information technology has replaced workers in routine codifiable tasks, it has magnified the value of social skills that allow workers to specialize and collaborate more efficiently. In a related vein, Gaetano Basso, Giovanni Peri, and Ahmed Rahman provide evidence that low-education US immigrants have helped blunt the impact of automation on native US workers.10
In work that appears prescient in light of the current pandemic, Nicholas Bloom, James Liang, John Roberts, and Zhichun Jenny Ying examine another labor market manifestation of advancing information technology: remote work.11 Partnering with a large Chinese travel agency, the researchers conduct a large field experiment in which travel agents were randomly offered the option to work from home. Among those offered the work-from-home option, both productivity and worker satisfaction rose. Ironically, promotion rates conditional on performance fell among those working from home, suggesting that not being in the office may also have hidden private costs.
This growing body of theory and evidence on labor market consequences of automation highlights an enduring macroeconomic puzzle raised by Robert Solow: “You can see the computer age everywhere but in the productivity statistics.”12 Though Solow’s observation dates to 1987, the puzzle has only deepened since that time — particularly with the pronounced slowdown in measured productivity growth in industrialized countries that Chad Syverson documents took place after approximately 2004.13 If machines are becoming so much cheaper and faster at accomplishing tasks once requiring expensive labor, why isn’t productivity rising more rapidly? Papers by Erik Brynjolfsson, Daniel Rock, and Syverson,14 among others, confront this puzzle, arguing that these productivity gains are near at hand. Their work makes the case that the productivity benefits of new technologies are masked by substantial unmeasured complementary investments made by technology adopters, such as new business processes and business models, novel products, and new human capital. If this explanation is correct, productivity should surge when the unmeasured investment phase slows and these hidden investments begin yielding measurable returns. Alternatively, Acemoglu and Restrepo offer a more skeptical interpretation of the productivity paradox, arguing that many heavily hyped information technologies are barely cheaper or more productive than the labor-using tasks that they displace.15 These “so-so” technologies, as these researchers label them, have the dual disadvantage of generating substantial worker displacement without yielding much of a productivity payoff. It is premature to know which view of our productivity predicament is correct.
Discrimination and Segregation in the Labor Market
A large body of recent scholarship by Labor Studies researchers brings new ambition, depth, and nuance to research on race and ethnicity in the labor market. Approximately 50 studies have focused specifically on race, discrimination, or segregation. In two studies, Marianne Bertrand and Esther Duflo16 and David Neumark17 review and synthesize the growing set of experimental analyses of discrimination.
A number of important articles have harnessed new data sources and state-of-the-art econometric methods to document new facts about racial and ethnic disparities in the labor market, and differences in economic mobility. A common thread in these studies is the degree of persistence in racial and ethnic gaps in economic and social outcomes. Economic gaps have remained immutable since the mid-20th century and have not been closed through individual or intergenerational economic mobility.
Patrick Bayer and Kerwin Kofi Charles decompose changes in the Black-White earnings gap between 1940 and 2010 into parts attributable to changes in the overall wage structure and to changes in the relative ranks of Blacks and Whites in the earnings distribution.18 They show that while the median White-Black male earnings gap declined between 1940 and 1970, it has grown substantially in recent decades and was at 1950s levels by the Great Recession. This growth in the gap has been driven by declining labor force participation, and in particular mass incarceration. The position of median Black workers in the White distribution of earnings has hardly improved since 1940, while there have been positional gains for Black workers in the 90th percentile of the earnings distribution.
These conclusions are echoed in Randall Akee, Maggie Jones, and Sonya Porter’s work using US tax records to document persistent differences in income shares across the entire income distribution between White households and Black, Native American, and Hispanic households.19 These differences are highly persistent. One of the breakthroughs in this paper is that by using the universe of tax returns, they can home in on small groups that previously could not be analyzed easily using survey data, notably Native Americans. Raj Chetty, Nathaniel Hendren, Jones, and Porter build on this work by computing measures of intergenerational mobility by race and ethnic group.20 They find that there has been very limited upward economic mobility of Black Americans and Native Americans, resulting in persistent gaps relative to White Americans over generations. On the other hand, Hispanic Americans have higher intergenerational mobility rates, leading to a convergence in the income gap between them and non-Hispanic Whites across generations.
One of the major themes in the studies on race and ethnicity is discrimination. Economics research has grappled with the topic of racial discrimination at least since Gary Becker’s seminal 1957 treatise on this topic.21 Research in this area has evolved from viewing discrimination as a specific action or transaction (e.g., a biased hiring decision) to a process that affects skills investment, information acquisition and inference, and self-perception, and even directly influences the productivity of the targets of discrimination.
Economists have historically categorized discrimination into two buckets: taste-based discrimination based upon animus (per Becker) and statistical discrimination based upon rational (Bayesian) information forecasting in the face of uncertainty about productivity (per Kenneth Arrow and Edmund Phelps). Recent research underscores why these categories are incomplete and, in some cases, not entirely coherent. Studying the productivity of cashiers in a French grocery store chain, Dylan Glover, Amanda Pallais, and William Pariente show that non-White cashiers perform on average significantly better than do White workers.22 Yet when assigned to managers who exhibit greater bias, the productivity of non-White workers — measured by absences and throughput — falls. This work calls into question the canonical assumption that discrimination represents unequal treatment for given expected levels of productivity by showing that prejudice can directly affect productivity.
An equally central assumption in the classic statistical discrimination literature is that employers hold rational expectations about worker capabilities, so that disparate treatment of minority and nonminority workers reflects unbiased but imprecise assessments of expected productivity. Challenging this view, J. Aislinn Bohren, Kareem Haggag, Alex Imas, and Devin G. Pope review evidence that statistical discrimination is often rooted in inaccurate information, such as bad statistics or stereotypes, which may of course emanate from prejudiced information sources.23 This observation is potentially critical for interpreting and redressing discrimination in practice. An employer that makes otherwise statistically sound decisions based on biased information may generate outcomes that are indistinguishable from animus-based discrimination. Yet the appropriate remedy might be to provide accurate information rather than to redress or punish bias. Consistent with a potential role for misinformation, Amanda Y. Agan and Sonja B. Starr show that employers located in neighborhoods with fewer Black residents appear much likelier to stereotype Black applicants as potentially criminal when they lack criminal record information.24
Many other studies provide fresh insights on discrimination. Experimental work by Joanna N. Lahey and Douglas R. Oxley shows how discrimination affects not only beliefs of potential employers but also the amount of attention that they devote to applicants from different race, gender, and age groups.25 In a resume audit study, Patrick M. Kline and Christopher R. Walters develop new tools for detecting the presence of employer discrimination.26
In an innovative experiment, Samantha Bielen, Wim Marneffe, and Naci H. Mocan experimentally manipulate the apparent race of defendants in recorded criminal trials using virtual reality tools. Law students, economics students, practicing lawyers, and judges who are randomly assigned to watch the trials are more likely to recommend conviction of defendants when they are portrayed in virtual reality as minorities.27
Benjamin Feigenberg and Conrad Miller reanalyze the classic question of whether there is an equity/efficiency tradeoff in policing activity, specifically in the case of motor vehicle searches.28 A tradeoff might arise if police are more effective in identifying offenders when permitted to use racial or ethnic profiling to select targets. The obvious cost of that approach is that members of disadvantaged groups — the vast majority of whom are not engaged in illicit conduct — would bear a disproportionate burden of police scrutiny. While models of statistical discrimination imply that this tradeoff exists in theory, Feigenberg and Miller find no such tradeoff in practice, at least in the case of vehicle searches conducted by Texas Highway Patrol troopers. The reason is that search rates by troopers are unrelated to the proportion of searches that detect illicit activity. By implication, the Texas Highway Patrol could equalize search rates across racial groups while increasing search yield without changing the total number of searches conducted.
Three papers look at historical episodes of discrimination in 20th century American history. Lisa D. Cook, Jones, David Rosé, and Trevon D. Logan document racial discrimination in public accommodations during the Jim Crow era.29 They provide new facts on how the prevalence of nondiscriminatory establishments varied by region; how they were far more likely to be located in redlined neighborhoods within cities; and how their prevalence was positively correlated with measures of material well-being and overall economic activity. Andreas Ferrara and Price V. Fishback show that German immigrants residing in the United States during World War I faced significant anti-German sentiment, particularly in counties with high wartime casualty rates where local newspapers published more anti-German slurs.30 German immigrants living in these counties were more likely to relocate; those who fled — and the counties that lost them — saw lower incomes for the next several decades.
Anna Aizer, Ryan Boone, Adriana Lleras-Muney, and Jonathan Vogel document beneficial effects of WWII defense production contracts in closing racial wage gaps.31 In particular, when the federal government awarded wartime production contracts to private firms, Black men in the surrounding metropolitan area were able to move into higher-skilled occupations, generating sizable and enduring earnings benefits. A key figure from their paper is reproduced as Figure 2.
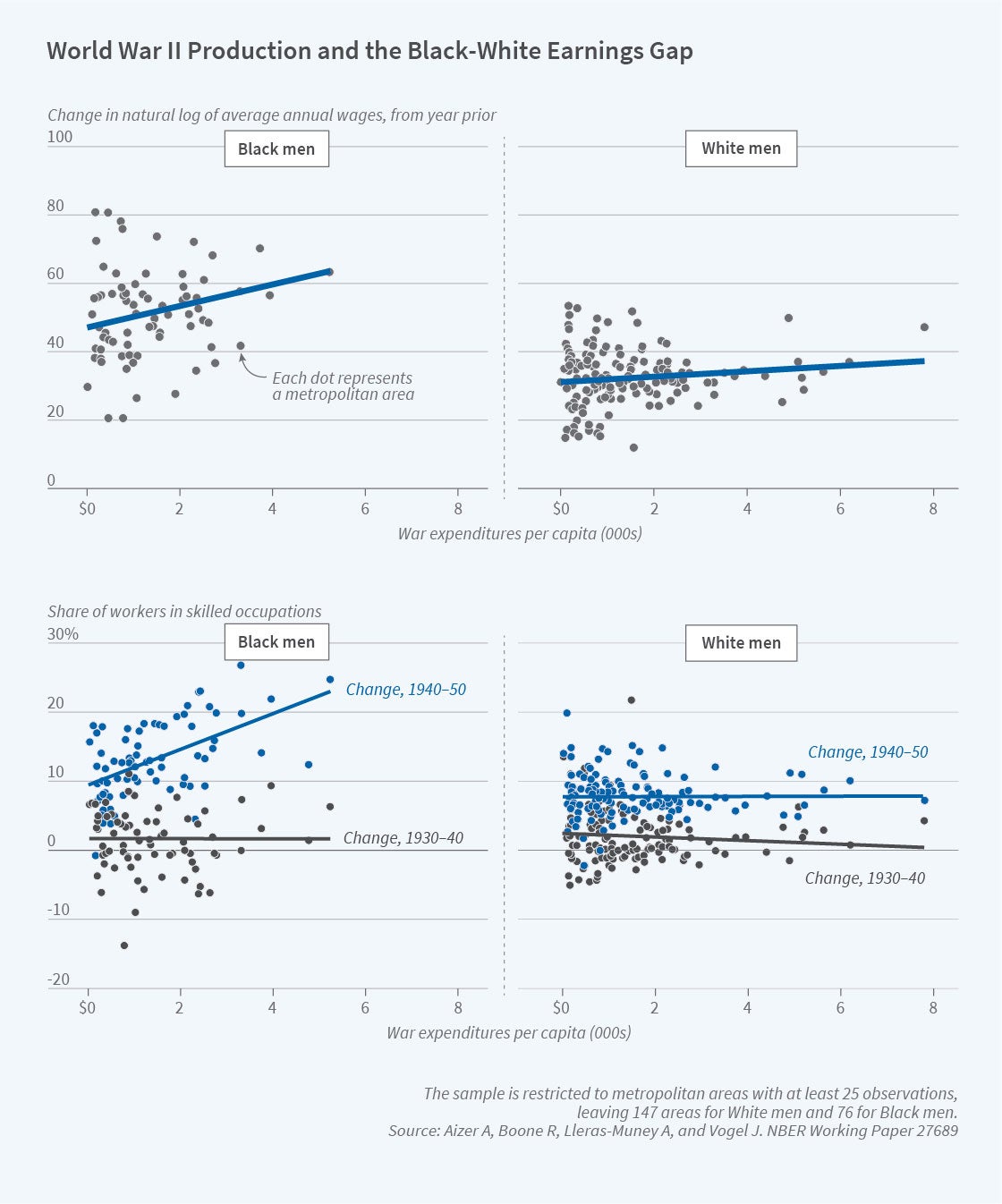
An increasingly prominent topic is whether the use of computerized algorithms for high-stakes decisions — such as which candidates receive interviews, which borrowers are granted loans, which defendants are released on bail — introduces the potential for algorithmic discrimination. Danielle Li, Lindsey R. Raymond, and Peter Bergman argue that machine learning algorithms that perform candidate selection for job interviews tend to reinforce past patterns of hiring by seeking candidates who are similar to those previously hired.32 The researchers show the downside to this approach by building a resume screening algorithm that values exploration — that is, sampling from diverse pools — as well as past practice. Using personnel data from a large firm, they show that this approach improves the quality of candidates selected for an interview, as measured by eventual hiring rates, while also increasing demographic diversity relative to the firm’s existing practices. A number of other studies develop tools for detecting bias in algorithms33,34 and consider how algorithms can be used more effectively.35
Minimum Wage
One of the great debates in labor economics has been on the effects of minimum wages on workers and firms. Over the last 10 years, a series of studies by Labor Studies affiliates has advanced this literature considerably. These studies have used new sources of variation in minimum wages from state and local minimum wage laws, new data sources, and new econometric approaches. Studies don’t always reach the same conclusions, likely owing to the different settings, time periods, and methodologies being used. Below we summarize several studies that look at employment and hour margins and that draw disparate conclusions.
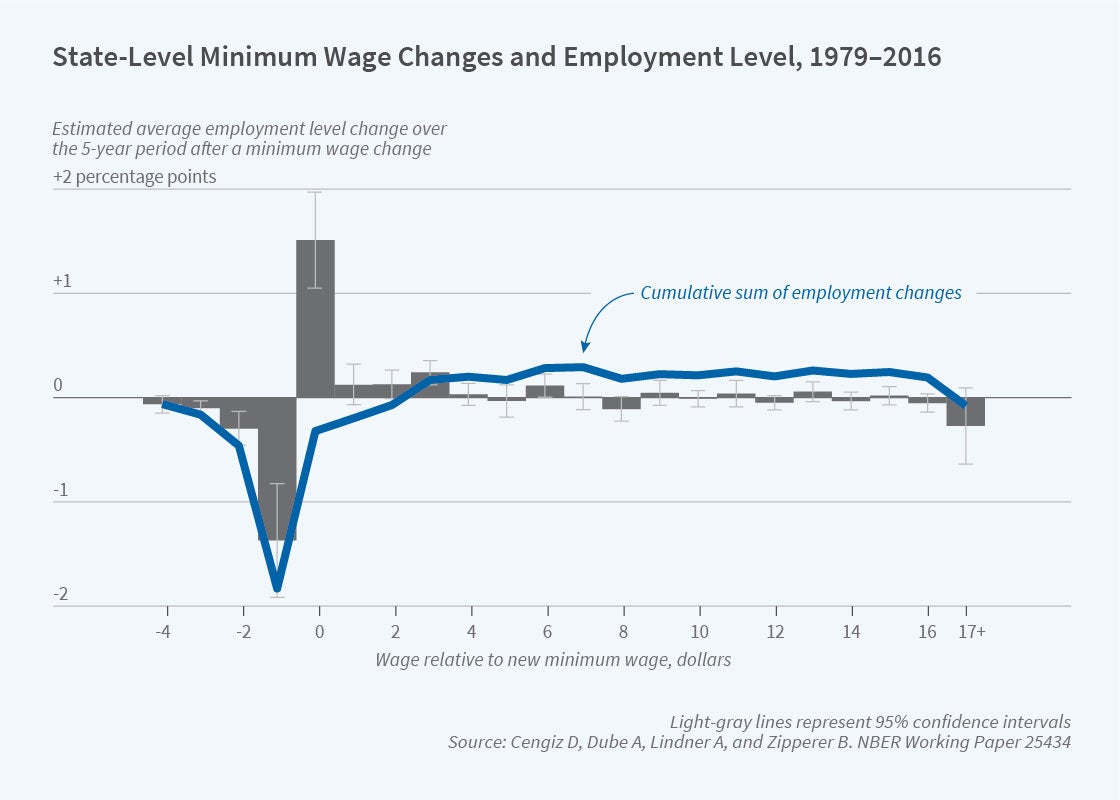
Jeffrey Clemens and Michael Wither examine the last increase (at the time of this writing) of the federal minimum wage, which took place over 2008–09.36 The timing of this increase makes this an especially interesting case since it coincided with the Great Recession, when the labor market may have been more sensitive to wage increases, but it also presents challenges for estimation, as it requires the researchers to carefully control for the business cycle. Using several approaches, including differences in how binding the minimum wage was between states, they estimate that employment fell by 8 percent among workers whose wages before the minimum wage increase were below the new minimum.
Ekaterina Jardim, Mark C. Long, Robert Plotnick, Emma van Inwegen, Jacob Vigdor, and Hilary Wething evaluate a minimum-wage ordinance that raised the minimum wage from $9.47 to $13 in Seattle.37 They use high-quality administrative data from the state of Washington that, unlike many other employer-employee matched datasets, include hours of work. Looking at workers employed in low-wage jobs prior to the minimum wage increase, matched to a comparison group of similar workers who were not affected by the minimum wage, they find that the ordinance increased wages, reduced hours of employment, reduced turnover, and reduced the rate of new entries in the workforce.
Doruk Cengiz, Arindrajit Dube, Attila Lindner, and Ben Zipperer examine state-level variation in the minimum wage using a bunching estimator approach.38 They first use a differences-in-differences estimator to estimate the effect of state-level changes in the minimum wage on employment counts in $0.25 buckets around the old and new minimum wage. They then ask whether employment losses below the new minimum wage are offset by employment gains at the new minimum wage and above it (due to spillovers). They find that these are comparable. This result does not mean that no workers lost their jobs, since it remains possible that the minimum wage induced reallocation between firms.39 However, their conclusion is that in the aggregate there were no significant losses.
As the literature on the minimum wage has evolved, researchers have explored new outcomes and more nuanced margins of adjustment to these policies, such as crime, infant and worker health, family income, and job search effort. Labor Studies researchers have examined the role of the minimum wage in economic, social, and health outcomes such as crime,40 criminal recidivism,41 infant health,42 worker health,43 family income,44 automation,45 and job search effort.46
Imperfect Competition and Labor Market Concentration
A major theme of Labor Studies researchers has been to test, quantify, and explore the implications of imperfect competition in the labor market. Two broad categories of studies on this topic have been to quantify the firm component of a worker’s pay and to test models of monopsony. Less common, but equally valuable, are studies that show direct evidence on imperfect competition, like the anti-competitive behavior found by Alan Krueger and Orley Ashenfelter among major franchisor employers who used “no-poaching of workers agreements.”47
Since the seminal work of John Abowd, Francis Kramarz, and David N. Margolis in 1994,48 a growing body of work has sought to measure firm differences in earnings and wages. Evidence that firms pay identical workers different wages is a violation of the law of one price and evidence of imperfect competition in the labor market. Over the last 10 years, there have been numerous studies on firm pay policies, due in part to the availability of large administrative datasets, increased computing power, and more efficient estimation approaches following the influential work of David Card, Jörg Heining, and Patrick Kline.49 A recent focus has been on developing new econometric approaches to correct biases that arise due to limited mobility of workers between firms. Some researchers, such as Stéphane Bonhomme, Kerstin Holzheu, Thibaut Lamadon, Elena Manresa, Magne Mogstad, and Bradley Setzler, have argued that the firm component of pay is less important after taking these biases into account,50 while others find a more important role for firms as well as evidence of positive sorting between high wages and high-wage firms when appropriately correcting estimates for sampling error.51 Jae Song, David Price, Fatih Guvenen, Bloom, and Till von Wachter find that changes in the allocation of workers across firms had a substantial role in the rise in earnings inequality between 1978 and 2013.52 Over this period, high-wage workers increasingly sorted into high-wage firms and also increasingly worked with one another. These two trends accounted for two-thirds of the rise in inequality over this period. Notably, the dispersion of firm-specific pay premiums did not increase; rather, workers who earned high wages elsewhere increasingly clustered at firms that paid larger premiums.
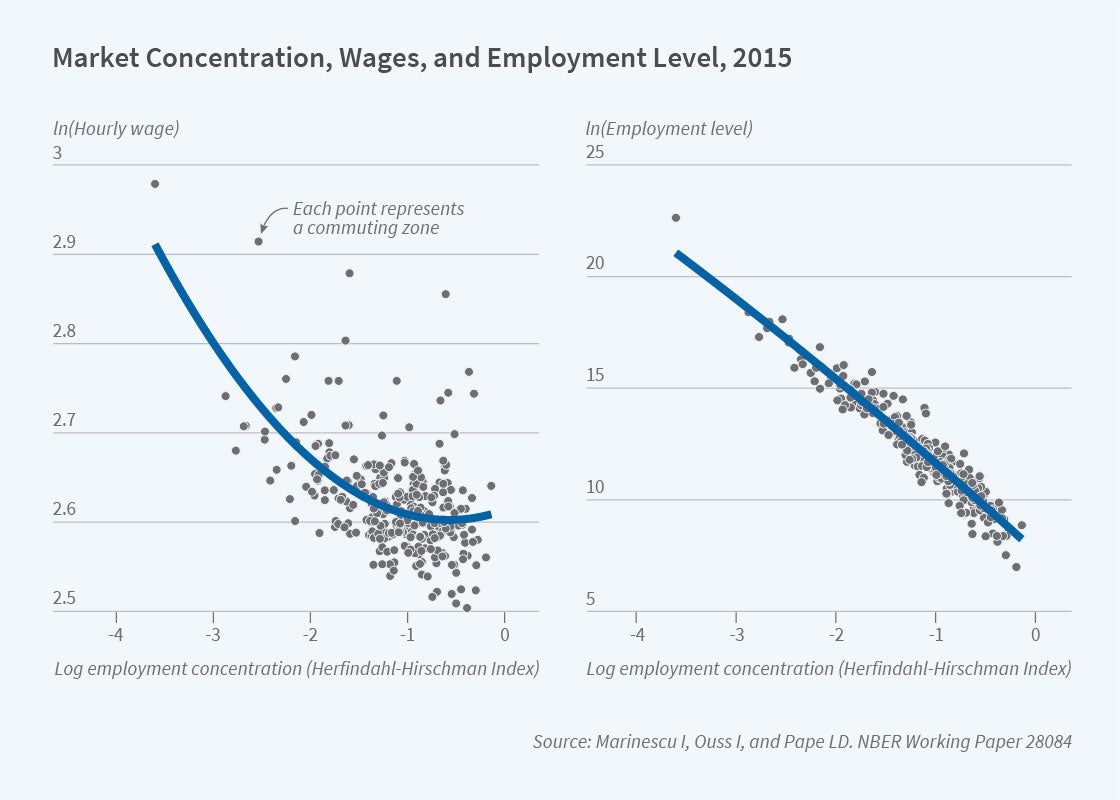
A related literature has sought to test the predictions of monopsonistic competition in the labor market. One class of studies has sought to compute measures of labor market concentration. For example, Ioana Marinescu, Ivan Ouss, and Louis-Daniel Pape calculate labor market concentration measures at the occupation and commuting zone levels in France and find that a 10 percent increase in concentration reduces wages of new hires by 0.9 percent.53 Their findings accord with other studies that take a similar approach.54 55 Matthew Kahn and Joseph Tracy note that geographic variation in market power will affect housing rents in a spatial equilibrium model.56 They find support for this prediction. A second class of monopsony studies seeks to directly estimate a necessary condition of the monopsony model, which is that the labor supply curve facing the firm is upward sloping. Kory Kroft, Yao Luo, Mogstad, and Setzler use the results from procurement auctions as shocks to a firm’s demand curve to effectively trace an upward-sloping labor supply relationship as they observe both employment and average labor earnings increasing following auction wins.57 Austan Goolsbee and Syverson estimate an upward-sloping labor supply curve in higher education institutions using school-specific labor demand instruments.58 A third class of studies59 estimates the negative relationship between wages and separation rates and uses that relationship to quantify the implied elasticity of labor supply facing the firm in a dynamic monopsony model.
The COVID-19 pandemic has resulted in historic disruptions in labor markets and has drawn new attention to many issues that have been long-standing topics of research in the Labor Studies Program. Assessing the impact of closures of nonessential businesses, of emergency relief programs for workers and firms, of potentially transformative changes in the geography of work, and of many other extraordinary developments over the past year will be an active subject of prospective research. The lessons of this research will guide future policy in response to economic shocks, and will provide new insights on the basic functioning of labor markets.
Endnotes
“Robots and Jobs: Evidence from US Labor Markets,” Acemoglu D, Restrepo P. NBER Working Paper 23285, March 2017.
“From Immigrants to Robots: The Changing Locus of Substitutes for Workers,” Borjas G, Freeman R. NBER Working Paper 25438, January 2019.
“Do Recessions Accelerate Routine-Biased Technological Change? Evidence from Vacancy Postings,” Hershbein B, Kahn L. NBER Working Paper 22762, October 2016.
“COVID-19 and Implications for Automation,” Chernoff A, Warman C. NBER Working Paper 27249, July 2020.
“Who Needs a Fracking Education? The Educational Response to Low-Skill Biased Technological Change,” Cascio E, Narayan A. NBER Working Paper 21359, February 2019.
“Robots Are Us: Some Economics of Human Replacement,” Benzell S, Kotlikoff L, LaGarda G, Sachs J. NBER Working Paper 20941, October 2018.
“Artificial Intelligence and Its Implications for Income Distribution and Unemployment,” Korinek A, Stiglitz J. NBER Working Paper 24174, December 2017.
“Demography, Unemployment, Automation, and Digitalization: Implications for the Creation of (Decent) Jobs, 2010–2030,” Bloom D, McKenna M, Prettner K. NBER Working Paper 24835, July 2018.
“The Growing Importance of Social Skills in the Labor Market,” Deming D. NBER Working Paper 21473, June 2017.
“Computerization and Immigration: Theory and Evidence from the United States,” Basso G, Peri G, Rahman A. NBER Working Paper 23935, October 2018.
“Does Working from Home Work? Evidence from a Chinese Experiment,” Bloom N, Liang J, Roberts J, Ying Z. NBER Working Paper 18871, March 2013.
“Challenges to Mismeasurement Explanations for the US Productivity Slowdown,” Syverson C. NBER Working Paper 21974, February 2016.
“The Productivity J-Curve: How Intangibles Complement General Purpose Technologies,” Brynjolfsson E, Rock D, Syverson C. NBER Working Paper 25148, October 2018.
“Automation and New Tasks: How Technology Displaces and Reinstates Labor,” Acemoglu D, Restrepo P. NBER Working Paper 25684, March 2019.
“Field Experiments on Discrimination,” Bertrand M, Duflo E. NBER Working Paper 22014, February 2016.
“Experimental Research on Labor Market Discrimination,” Neumark D. NBER Working Paper 22022, February 2016.
“Divergent Paths: Structural Change, Economic Rank, and the Evolution of Black-White Earnings Differences, 1940–2014,” Bayer P, Charles K. NBER Working Paper 22797, September 2017.
“Race Matters: Income Shares, Income Inequality, and Income Mobility for All US Races,” Akee R, Jones M, Porter S. NBER Working Paper 23733, August 2017.
“Race and Economic Opportunity in the United States: An Intergenerational Perspective,” Chetty R, Hendren N, Jones M, Porter S. NBER Working Paper 24441, December 2019.
“Discrimination as a Self-Fulfilling Prophecy: Evidence from French Grocery Stores,” Glover D, Pallais A, Pariente W. NBER Working Paper 22786, October 2016.
“Inaccurate Statistical Discrimination: An Identification Problem,” Bohren J, Haggag K, Imas A, Pope D. NBER Working Paper 25935, July 2020.
“Employer Neighborhoods and Racial Discrimination,” Agan A, Starr S. NBER Working Paper 28153, November 2020.
“Discrimination at the Intersection of Age, Race, and Gender: Evidence from a Lab-in-the-Field Experiment,” Lahey J, Oxley D. NBER Working Paper 25357, December 2018.
“Reasonable Doubt: Experimental Detection of Job-Level Employment Discrimination,” Kline P, Walters C. NBER Working Paper 26861, August 2020.
“Racial Bias and In-Group Bias in Judicial Decisions: Evidence from Virtual Reality Courtrooms,” Bielen S, Marneffe W, Mocan N. NBER Working Paper 25355, May 2019.
“Racial Disparities in Motor Vehicle Searches Cannot Be Justified by Efficiency,” Feigenberg B, Miller C. NBER Working Paper 27761, August 2020.
“The Green Books and the Geography of Segregation in Public Accommodations,” Cook L, Jones M, Rosé D, Logan T. NBER Working Paper 26819, March 2020.
“Discrimination, Migration, and Economic Outcomes: Evidence from World War I,” Ferrara A, Fishback P. NBER Working Paper 26936, April 2020.
“Discrimination and Racial Disparities in Labor Market Outcomes: Evidence from WWII,” Aizer A, Boone R, Lleras-Muney A, Vogel J. NBER Working Paper 27689, August 2020.
“Hiring as Exploration,” Li D, Raymond L, Bergman P. NBER Working Paper 27736, August 2020.
“Racial Bias in Bail Decisions,” Arnold D, Dobbie W, Yang C. NBER Working Paper 23421, May 2017.
“Measuring Racial Discrimination in Bail Decisions,” Arnold D, Dobbie W, Hull P. NBER Working Paper 26999, October 2020.
“Human Decisions and Machine Predictions,” Kleinberg J, Lakkaraju H, Leskovec J, Ludwig J, Mullainathan S. NBER Working Paper 23180, February 2017.
“The Minimum Wage and the Great Recession: Evidence of Effects on the Employment and Income Trajectories of Low-Skilled Workers,” Clemens J, Wither M. NBER Working Paper 20724, December 2014.
“Minimum Wage Increases and Individual Employment Trajectories,” Jardim E, Long M, Plotnick R, van Inwegen E, Vigdor J, Wething H. NBER Working Paper 25182, October 2018.
“The Effect of Minimum Wages on Low-Wage Jobs: Evidence from the United States Using a Bunching Estimator,” Cengiz D, Dube A, Lindner A, Zipperer B. NBER Working Paper 25434, January 2019.
“Survival of the Fittest: The Impact of the Minimum Wage on Firm Exit,” Luca D, Luca M. NBER Working Paper 25806, May 2019.
“Do Minimum Wage Increases Reduce Crime?” Fone Z, Sabia J, Cesur R. NBER Working Paper 25647, November 2020.
“The Minimum Wage, EITC, and Criminal Recidivism,” Agan A, Makowsky M. NBER Working Paper 25116, September 2018.
“Effects of the Minimum Wage on Infant Health,” Wehby G, Dave D, Kaestner R. NBER Working Paper 22373, March 2018.
“Do Minimum Wage Increases Influence Worker Health?” Horn B, Maclean J, Strain M. NBER Working Paper 22578, August 2016.
“Minimum Wages and the Distribution of Family Incomes,” Dube A. NBER Working Paper 25240, November 2018.
“People versus Machines: The Impact of Minimum Wages on Automatable Jobs,” Lordan G, Neumark D. NBER Working Paper 23667, January 2018.
“The Minimum Wage and Search Effort,” Adama C, Meer J, Sloan C. NBER Working Paper 25128, February 2021.
“Theory and Evidence on Employer Collusion in the Franchise Sector,” Krueger A, Ashenfelter O. NBER Working Paper 24831, July 2018.
“High Wage Workers and High Wage Firms,” Abowd J, Kramarz F, Margolis D. NBER Working Paper 4917, November 1994.
“Workplace Heterogeneity and the Rise of West German Wage Inequality,” Card D, Heining J, Kline P. NBER Working Paper 18522, November 2012.
“How Much Should We Trust Estimates of Firm Effects and Worker Sorting?” Bonhomme S, Holzheu K, Lamadon T, Manresa E, Mogstad M, Setzler B. NBER Working Paper 27368, August 2020.
“Leave-out Estimation of Variance Components,” Kline P, Saggio R, Solvsten M. NBER Working Paper 26244, September 2019.
“Firming Up Inequality,” Song J, Price D, Guvenen F, Bloom N, von Wachter T. NBER Working Paper 21199, June 2015.
“Wages, Hires, and Labor Market Concentration,” Marinescu I, Ouss I, Pape L. NBER Working Paper 28084, November 2020.
“Labor Market Concentration,” Azar J, Marinescu I, Steinbaum M. NBER Working Paper 24147, February 2019.
“Strong Employers and Weak Employees: How Does Employer Concentration Affect Wages?” Benmelech E, Bergman N, Kim H. NBER Working Paper 24307, February 2018.
“Monopsony in Spatial Equilibrium,” Kahn M, Tracy J. NBER Working Paper 26295, June 2020.
“Imperfect Competition and Rents in Labor and Product Markets: The Case of the Construction Industry,” Kroft K, Luo Y, Mogstad M, Setzler B. NBER Working Paper 27325, August 2020.
“Monopsony Power in Higher Education: A Tale of Two Tracks,” Goolsbee A, Syverson C. NBER Working Paper 26070, July 2019.
“Monopsony in Movers: The Elasticity of Labor Supply to Firm Wage Policies,” Bassier I, Duba A, Naidu S. NBER Working Paper 27755, August 2020.