Mechanisms and Impacts of Innovation Policy
The importance of innovation to job creation and economic growth — especially in young, high-growth firms — is widely accepted among economists as well as members of the business and policy communities. There is also a recognition that, at least at some times or in certain settings, the private sector underinvests in innovation, creating an opportunity for the public sector to step into the breach.
The longstanding problem is how. What tools are most effective?
There are myriad opportunities for government programs to fail. For example, if a program subsidizes only the “best projects,” those that would likely have gone forward with private capital regardless of government involvement, this is likely to be a poor use of taxpayer dollars. Alternatively, if only poor-quality projects are supported, they might fail even with government support.
In my research, I seek to understand the effects of, and mechanisms behind, common policy tools that subsidize high-growth entrepreneurship and innovation in the United States. In doing so, I hope to inform policymaking and shed light on the constraints and trade-offs of the innovation process.
Three key themes emerge in my work. First, program design appears to be more important than the amount of funding. For example, it is important to enable innovators to pivot and to control the commercialization pathway of their ideas. Second, effectiveness depends on which firms decide to apply for support. Programs need to target firms with the potential to benefit, and succeed in getting them to apply for support. Finally, direct federal funding plays an important role in our innovation ecosystem and is not always substitutable with private or privately intermediated alternatives.
The Evaluation Challenge
Economists have long been interested in evaluating government innovation programs, but it has been hard to identify causal effects. Program administrators are typically loath to run experiments. My work has addressed this challenge by employing several empirical approaches.
The most important of these methods is a regression discontinuity design (RDD) in which I compare winning and losing applicants within a competition for a grant or contract. I control for the rank that the program assigns to each applicant. Importantly, the cutoff decision determining which ranks win is exogenous to the ranking process. The key insight is that near the cutoff for winning, winners and losers should be similar, creating a natural experiment.
In other work, I use staggered program rollout designs, while addressing potential bias from pretreatment observations being considered by the model as controls. A final method is to instrument for funding using plausibly exogenous shocks. All three of these methods can be applied in many policy evaluation settings, and if carefully executed can reveal causal effects.
Design and Selection: Evidence from the SBIR Program
The US Small Business Innovation Research (SBIR) program, which was established in 1982, is the main vehicle by which the federal government directly supports innovation at small firms and encourages them to enter the federal contracting pipeline. It is available at 11 federal agencies and always has two stages. Firms first apply to a subsector- or topic-specific Phase 1 competition for awards, usually about $150,000. Phase 1 winners may then apply nine months later for $1 million Phase 2 awards. The SBIR program has been imitated around the world, and thus represents a particularly important research setting.
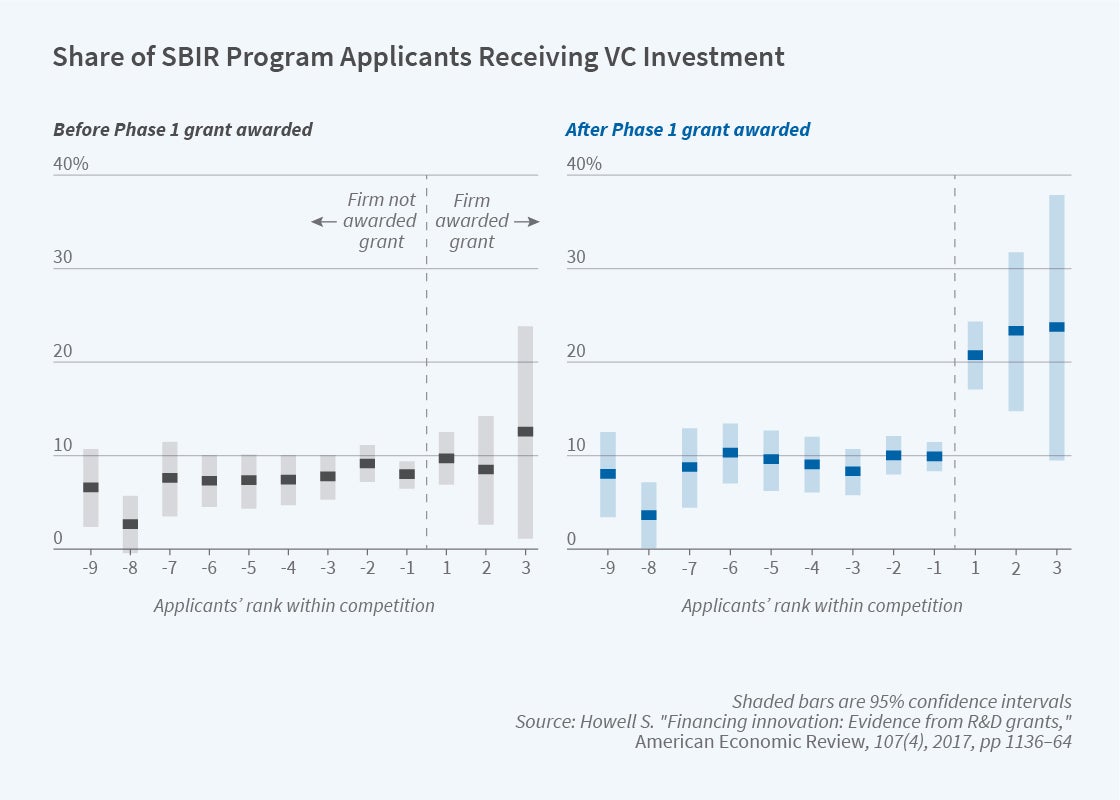
In a project using data from the SBIR program at the Department of Energy (DOE), I conducted the first quasi-experimental, large-sample evaluation of R&D grants to private firms.1 Using the RDD approach, I found strong effects of the Phase 1 awards: they dramatically increased citation-weighted patenting, the chance of raising venture capital (VC) investment, revenue, and survival. On average, the early-stage grants did not crowd out private capital and instead enabled new technologies to go forward.
The picture was not so rosy for Phase 2. This larger grant had no measurable effect, except for a small positive effect on citation-weighted patents. I found evidence of adverse selection in Phase 2 applications. Almost 40 percent of Phase 1 winners did not apply to Phase 2, and these were disproportionately VC recipients. Phase 2 eligibility criteria, which include requirements that the firm not change its business strategy and not be more than 50 percent investor owned, apparently generated this adverse selection. This finding underscores the general theme that who decides to apply —i.e., selection — is a powerful force determining the effectiveness of a program.
Selection also plays a role in my work with John Van Reenen, Jason Rathje, and Jun Wong, which explores the design of public sector innovation procurement initiatives.2 A key decision is whether to take a centralized approach where the desired innovation is tightly specified or to take a more open, decentralized approach where applicants are given leeway to suggest solutions. We compare these two approaches using a quasi-experiment conducted by the US Air Force SBIR program.
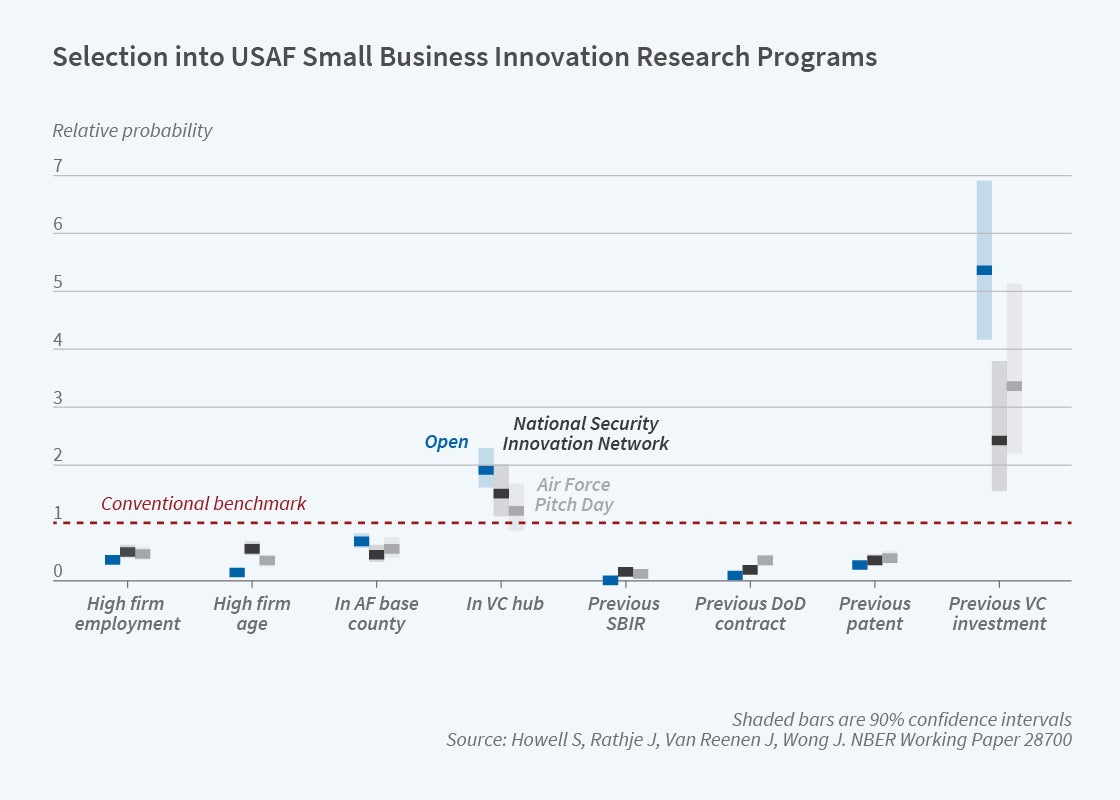
That program holds multiple competitions about every four months in which firms apply to develop military technologies. The Conventional Program approach is to hold competitions with highly specific topics such as “Affordable, Durable, Electrically Conductive Coating or Material Solution for Silver Paint Replacement on Advanced Aircraft.” After 2018, the Air Force also included an Open Program competition that ran alongside the Conventional model, wherein firms could propose anything they thought the Air Force would need.
We found that winning an open topic competition had positive and significant effects on three outcomes desired by the program administrators: the chances of the military adopting the new technology, the probability of subsequent VC investment, and patenting and patent originality. By contrast, winning a Conventional award had no measurable effect on any of these outcomes. Nor were there any causal impacts of winning a Conventional award between 2003 and 2017, before the Open Program was introduced.
Both selection and decentralization played a role in the Open Program’s success. It reached firms with startup characteristics that were less likely to have had previous defense contracts — a selection effect. At the same time, however, we also found that openness matters. For example, there were significantly more positive effects of Open awards even among the firms that applied to both the Open and Conventional Programs. Also, when a Conventional topic was less specific and thus closer to the Open Program’s approach, winning an award for that topic significantly increased innovation.
The Open Program seems to work in part because it provides firms with an avenue to identify technological opportunities of which the government is not yet fully aware, and it enables firms to pursue their private and government commercialization pathways simultaneously. These results are relevant beyond the Air Force, as governments and private firms increasingly turn to open or decentralized approaches to soliciting innovation.
Incentives: Who Is Funding?
I also found benefits of openness in a different setting: university research. Unlike the two projects focusing on important government programs, this project explored what happened when federal funding declined, shedding light on substitutability with private funding.
Together with Tania Babina, Alex He, Elisabeth Perlman, and Joseph Staudt, I asked whether declines in federal R&D funding affected the innovation outputs of academic research.3 We linked data on all employees of all grants at 22 universities to career outcomes of individuals in the US Census Bureau’s IRS W-2 files, patent inventors, and publication authors in the PubMed database.
We found that a negative federal funding shock nearly halved a researcher’s chance of founding a high-tech startup, but doubled their chance of being an inventor on a patent. The shock also reduced the number of publications, especially those that are more basic, more cited, and in higher-impact journals.
What could explain these seemingly puzzling findings? We found evidence that they were in part driven by a shift from federal to private funders. While federal awards typically assert no property rights to research outcomes, private firms have incentives to appropriate research outputs, and for that reason employ complex legal contracts with researchers. As the composition of research funding shifts from federal to private sources, outputs are more often commercialized by the private funder, rather than disseminated openly in publications or taken to a startup by the researcher.
In all the programs discussed thus far, the government directly targets the operating firm or innovator. A popular alternative approach is to target financial intermediaries, such as VC funds — as is done in Israel, Canada, Singapore, China, and some other countries — or angel investors.
More than 14 countries and most US states offer angel investor tax credits. Matthew Denes, Filippo Mezzanotti, Xinxin Wang, Ting Xu, and I studied these credits.4 They offer several promising features: no need for government to pick winners, low administrative burdens, and market incentives with investors retaining skin in the game.
Angel tax credits increase the number of angel investments by approximately 18 percent and the number of individual angel investors by 32 percent. Surprisingly, however, we found that angel tax credits do not appear to generate high-tech firm entry or job creation.
One reason for this outcome appears to be selection: additional investment flows to relatively low-growth firms. The angel investments appear to crowd out investment that would have happened otherwise, as common informal equity stakes — often made by insiders in the firm or family members of the entrepreneur — are labeled as “angel.”
Another reason emerges from the theory of investment in early stage, high-growth firms. These investments have fat-tailed returns. We find that as the right tail grows fatter, professional investors become less sensitive to the tax credits. This limits the ability of the policy to reach its intended targets — potentially high-growth startups. In the words of one survey respondent explaining why angel tax credits do not affect decision-making, “I’m more focused on the big win than offsetting a loss.”
Spillovers and Financial Constraints
Both the university research and angel tax credit projects highlight the role of decision-maker incentives, which determine the projects that get funded and their pathways to commercialization. While private funders and private intermediaries have attractive features, notably reducing the burden on government and costly taxpayer dollars, they have different incentive structures relative to government funders. In the programs I have studied, private sector actors have incentives to select projects with fewer knowledge spillovers.
My work also highlights that effective programs target financially constrained firms. The strong positive effects of the SBIR programs stem from awards to small, young firms that are new to SBIR and to government contracting. J. David Brown and I show that the small firms that benefit from SBIR awards use the funds in part to pay employees, especially those with long tenure at the firm.5 These financially constrained firms appear to finance themselves in part by engaging in back-loaded wage contracts with their workers. By alleviating constraints, an effective program paves the way for future investment and growth.
In contrast, in both the DOE and Air Force settings, it seems that SBIR awards crowd out private investment among larger firms that win many such awards. Similarly, angel tax credit programs crowd out private activity because investors often use them in deals that would have occurred regardless of the program.
While money is of course fungible, my research suggests that the source of innovation funds and program design — especially design features that affect who applies to the program — matter a great deal.
Endnotes
“Financing Innovation: Evidence from R&D Grants,” Howell S. American Economic Review 107(4), April 2017, pp. 1136–1164.
“Opening Up Military Innovation: Causal Effects of Reforms to US Defense Research,” Howell S, Rathje J, Van Reenen J, Wong J. NBER Working Paper 28700, July 2022.
“The Color of Money: Federal vs. Industry Funding of University Research,” Babina T, He A, Howell S, Perlman R, Staudt J. NBER Working Paper 28160, December 2020. Forthcoming in The Quarterly Journal of Economics as “Cutting the Innovation Engine: How Federal Funding Shocks Affect University Patenting, Entrepreneurship, and Publications.”
“Investor Tax Credits and Entrepreneurship: Evidence from US States,” Denes M, Howell S, Mezzanotti F, Wang X, Xu T. NBER Working Paper 27751, October 2021. Forthcoming in Journal of Finance.
“Do Cash Windfalls Affect Wages? Evidence from R&D Grants to Small Firms,” Howell S, Brown J. NBER Working Paper 26717, January 2020, and The Review of Financial Studies, October 2022.