The Economic Effects of Social Networks
Researchers have long understood that social interactions can shape many aspects of social and economic activity, including migration, trade, job-seeking, investment behavior, product adoption decisions, and social mobility.1 Traditionally, however, it has been challenging to analyze and quantify the economic effects of social interactions, in large part because of the absence of large-scale and representative data on social networks.
Over the past years, we have worked with deidentified data on social connections from Facebook to expand our understanding of the role of social networks across a large number of settings in economics and finance. Facebook is unique in its scale and coverage: at the end of 2020, the social network had 2.8 billion active users globally and 258 million active users in the United States and Canada, providing a rare opportunity to measure real-world social networks at population scale. Here we review some of our findings from this body of work, which uses both deidentified individual-level data and publicly available aggregated data on social connections between geographies.
Shaping Beliefs and Behaviors in the Housing Market
In a first series of papers, we studied the effect of social interactions in the housing market. In a paper with Michael Bailey and Rachel Cao, we showed that individuals are more likely to consider housing a good investment — and are in fact more likely to actually purchase a house — if their friends experienced larger recent house price increases.2
This project started from the observation that different people living in the same neighborhood can be exposed to very different housing market experiences through their social networks. Consider two neighbors living in New York, Amy and Ben. Amy has many friends who live in San Diego, a housing market that has been booming over the past decade, and therefore often hears her friends talk about rising house prices. Ben has more friends living in Chicago, which has seen much lower house price growth, and hears far fewer stories about fast house price growth. We investigated whether the different stories that Amy and Ben hear from their friends affected whether they considered buying a house in New York to be a good investment.
To measure people’s housing market beliefs, Facebook conducted an online survey of some of its users in Los Angeles. We found that individuals living in the same zip code often disagreed substantially in their expectations about future local house price growth. We then matched individuals’ survey responses to deidentified data on the location of their Facebook friends, and discovered that individuals with friends living in areas of the US where house prices had recently gone up were more optimistic about Los Angeles housing market investments than individuals with friends in parts of the country where house prices had not done so well. Importantly, all of this effect was concentrated among the subset of people — comprising about half of our sample — who had told us in the survey that they regularly talked with their friends about housing market developments.
After showing that friends’ house price experiences influence the way people perceive housing market investments, we investigated whether social interactions with their friends also affected people’s decisions to buy a house. We found that individuals whose friends experienced larger recent house price increases were more likely to transition from renting to owning. They also bought larger houses and paid more for a given house. These results highlight that individuals’ investment decisions are not made in a social vacuum. What they hear from their friends affects how attractive they perceive an asset to be, even if the experience of those friends arguably does not contain a lot of information that is relevant for the true valuation of the asset.
In follow-on work with Bailey and Eduardo Dávila, we showed that social interactions, through influencing housing market beliefs, also affect individuals’ leverage choices in the mortgage market.3 Specifically, we found that individuals whose friends had experienced recent house price declines — and who were thus more pessimistic — chose smaller down payments and higher leverage in an attempt to shield their savings from possible declines in house prices.
Peer Effects in Product Adoption
We next explored the role of social interactions for product adoption decisions, which can be affected by peers for a variety of reasons, for instance, because social interactions provide information or because of the importance of consumption externalities, such as a desire to keep up with the Joneses. Together with Bailey, Drew Johnston, and Arlene Wong, we studied how a new phone purchase by a friend affected a person’s own phone purchasing probability.4 We focused on purchases of new phones, since the Facebook data include information on device use from mobile-active users, allowing us to identify new phone purchases for an individual and her social network.
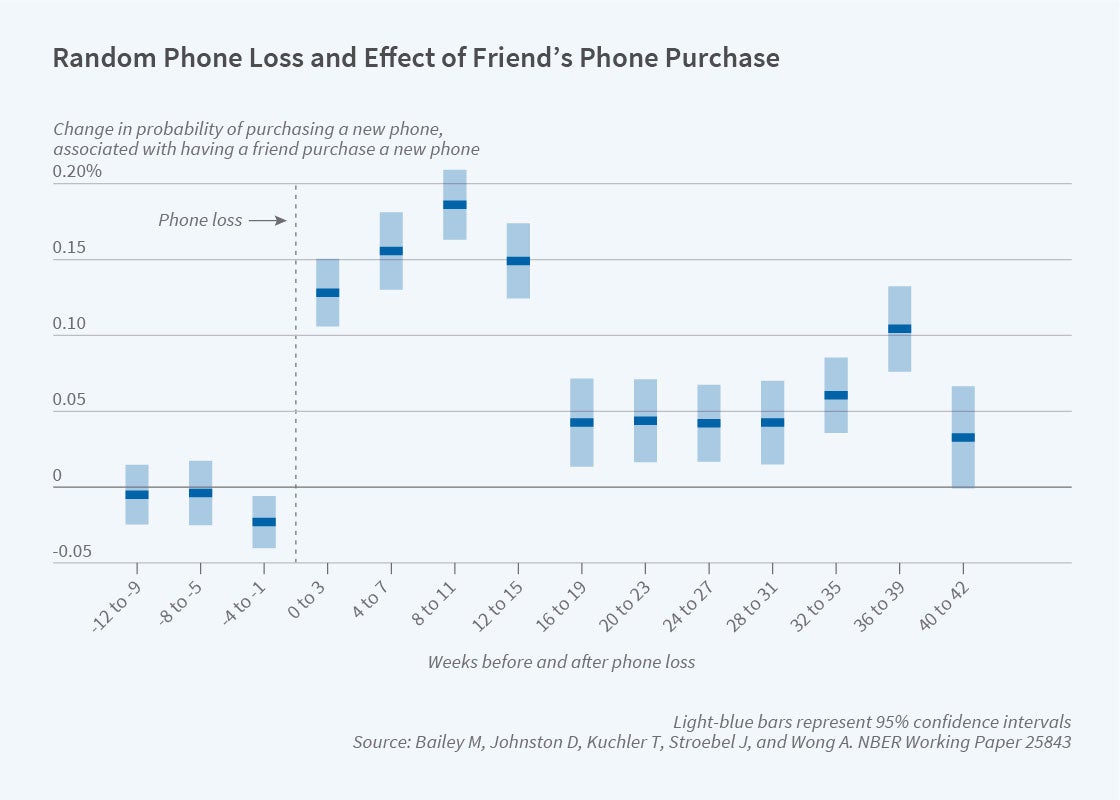
To separately identify the role of peer effects from common preferences and common interactions, we exploited variation in friends’ phone purchases after a random phone loss. We found that having a friend who purchased a new phone following such a random phone loss had a substantial and long-lasting effect on a person’s own probability of getting a new phone. Figure 1 shows the effect of having a friend purchase a new phone in prior and subsequent four-week periods. Quantitatively, having one extra friend purchase a new phone increases an individual’s own probability of purchasing a new phone over the next four months by 0.6 percentage points, relative to a baseline probability of buying a new phone over this horizon of about 14.6 percent.
We also found that positive peer effects were largest for the same device and brand purchased by the peer: when your friend buys a new iPhone, this primarily increases your own probability of buying an iPhone. In addition, we showed that some of these incremental same-brand purchases come at the expense of purchases from competing brands: some people who are induced by their friends to buy a new iPhone would have otherwise bought a Samsung Galaxy, while others would not have bought a new phone at all.
These across-brand demand spillovers highlight the important competitive implications for firms of peer effects: losing a customer to a competitor does not only mean missing out on positive peer effects that this customer could have had, but may also lead to future losses of other customers through competitive peer effects. Our evidence also suggests that social learning contributes substantially to the observed peer effects: when a friend purchases a new phone, their purchase allows the individual to learn about the features of the specific phone their friend purchased, making them more likely to buy that specific model.
Social Interactions and Public Health Behavior
More recently, we studied the effects of social interactions on behavior in the public health domain. Together with Bailey, Johnston, Martin Koenen, and Dominic Russel, we showed that social network exposure to COVID-19 cases shaped individuals’ beliefs and behaviors concerning the coronavirus.5 In particular, we showed that individuals with friends in areas with worse COVID-19 outbreaks reduced their mobility more than otherwise similar individuals with friends in less-affected areas. The effects on social distancing behavior are large and long-lasting. We also showed that individuals with higher friend-exposure to COVID-19 were more likely to publicly post in support of social distancing measures and less likely to be members of groups advocating reopening the economy. These findings suggest that friends can influence individuals’ beliefs about the risks of the disease and thereby induce them to engage in mitigating public health behavior.
The Social Connectedness Index
While our research working with deidentified individual-level data on social networks has documented the importance of social interactions across a number of important settings, many interesting outcome variables are not observed in the Facebook data. In the second strand of our research agenda, we therefore work with data on the geographic structure of social networks, which can be matched with outcomes of interest observed at various levels of geographic aggregation.
To facilitate such research, we worked with Bailey, Cao, and Wong to develop the Social Connectedness Index (SCI), a measure of the relative social connectedness between pairs of geographies.6 Formally, the SCI between two locations i and j is given by:
Here, FB_Users_i and FB_Users_j are the number of Facebook users in each location, and FB_Connections_(i,j) is the number of Facebook friendship connections between users in the two locations. The interpretation of the SCI is that if it is twice as large, a given Facebook user in i is about twice as likely to be connected with a given Facebook user in j.
The SCI has global coverage and is available at many levels of geographic aggregation, including between US counties and global subnational regions. (The SCI data can be downloaded for free and without usage restrictions from https://data.humdata.org/dataset/social-connectedness-index)
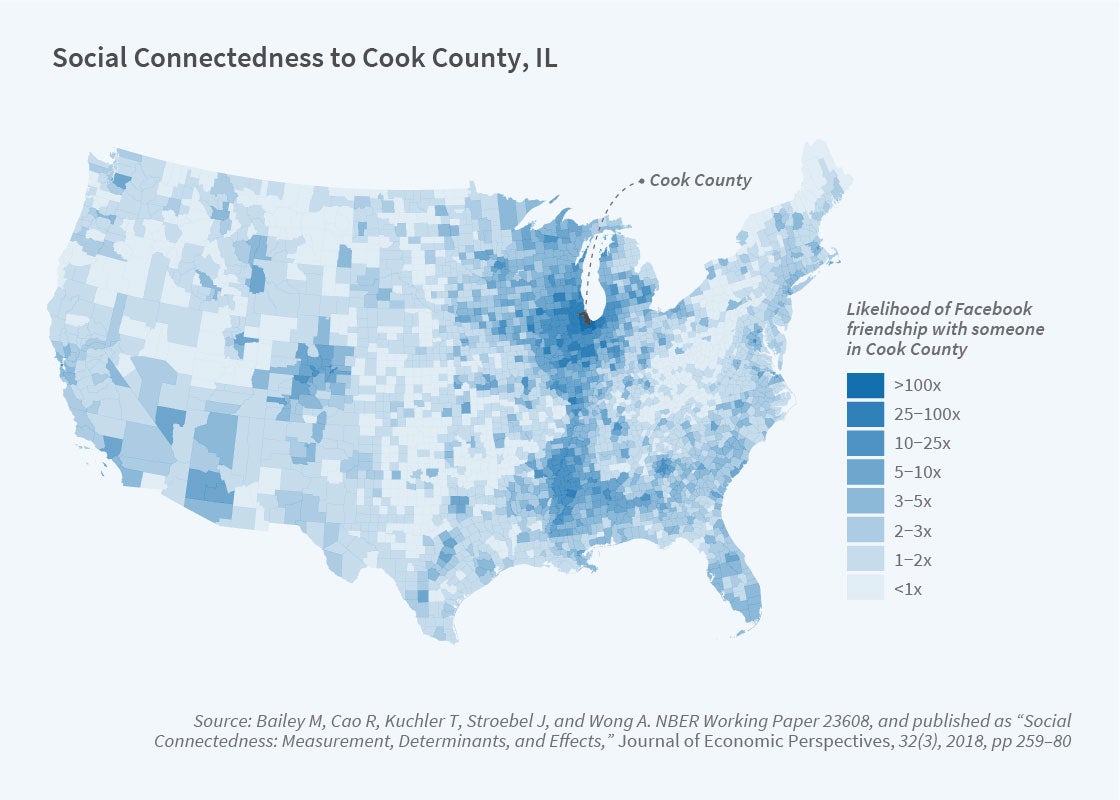
The SCI reveals many interesting patterns of social connectedness, such as the role of past migration flows. Panel A of Figure 2 shows the social connectedness to Cook County, Illinois — home to the city of Chicago — of other US counties. Darker shades correspond to stronger social connectedness. Besides the strong social connections to counties near Chicago, a salient pattern is the strong social links between Chicago and Southern counties around the Mississippi River. These connections capture present-day links caused by the Great Migration, the long-term movement of African Americans from the South to the urban North between 1916 and 1970.
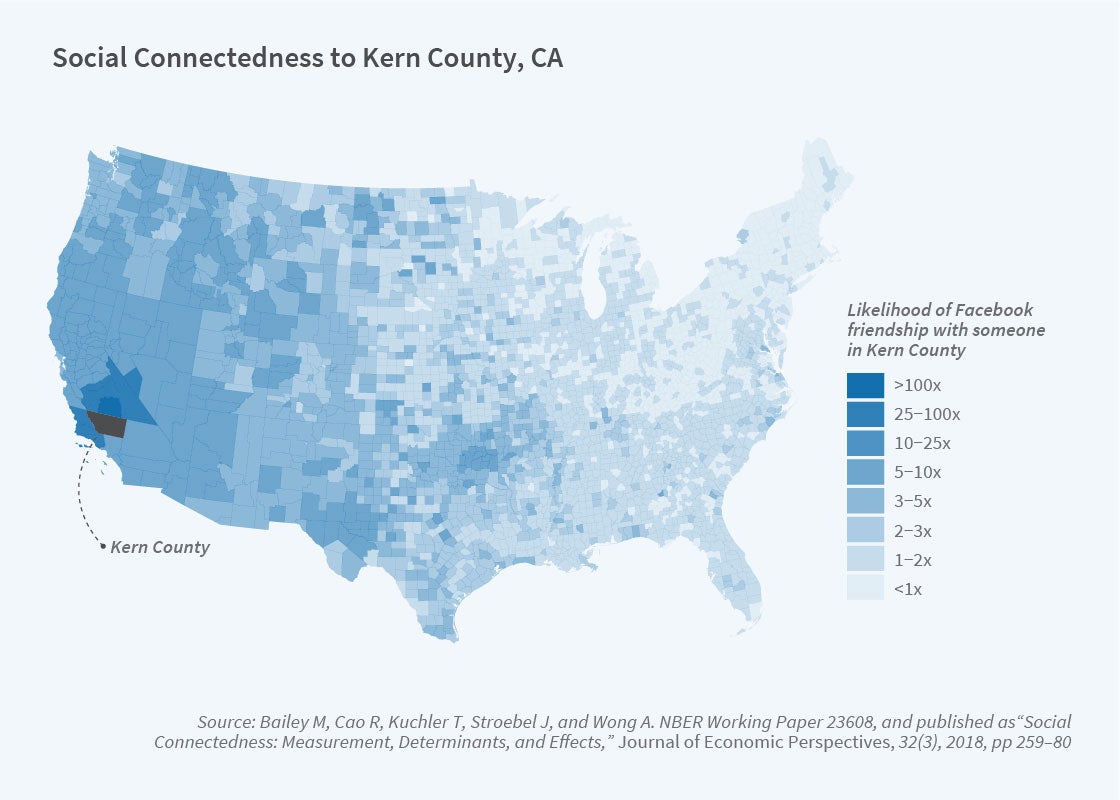
Similarly, Panel B of Figure 2 shows the social connections to Kern County, California, home to the city of Bakersfield. Again, past migration patterns show up strongly in shaping Kern County’s present-day social connectedness. A strong cluster of links between Kern County and Oklahoma is a testament to the long-lasting effects of the Dust Bowl migrants who fled Oklahoma in the 1930s for Bakersfield and other parts of the western United States. More recently, the strong links to McKenzie County, North Dakota, and surrounding counties are likely a result of the connections between oil workers from Kern County — a major oil-producing region — and workers in the Bakken oil fields.
The SCI also shows the effects of international migration patterns into the United States on present-day friendship links. An example: Figure 3 shows the social connectedness of US counties to Norway. There are strong social links to areas in Minnesota and Wisconsin, likely related to the large immigration of Norwegians to these states in the late 19th and early 20th centuries.
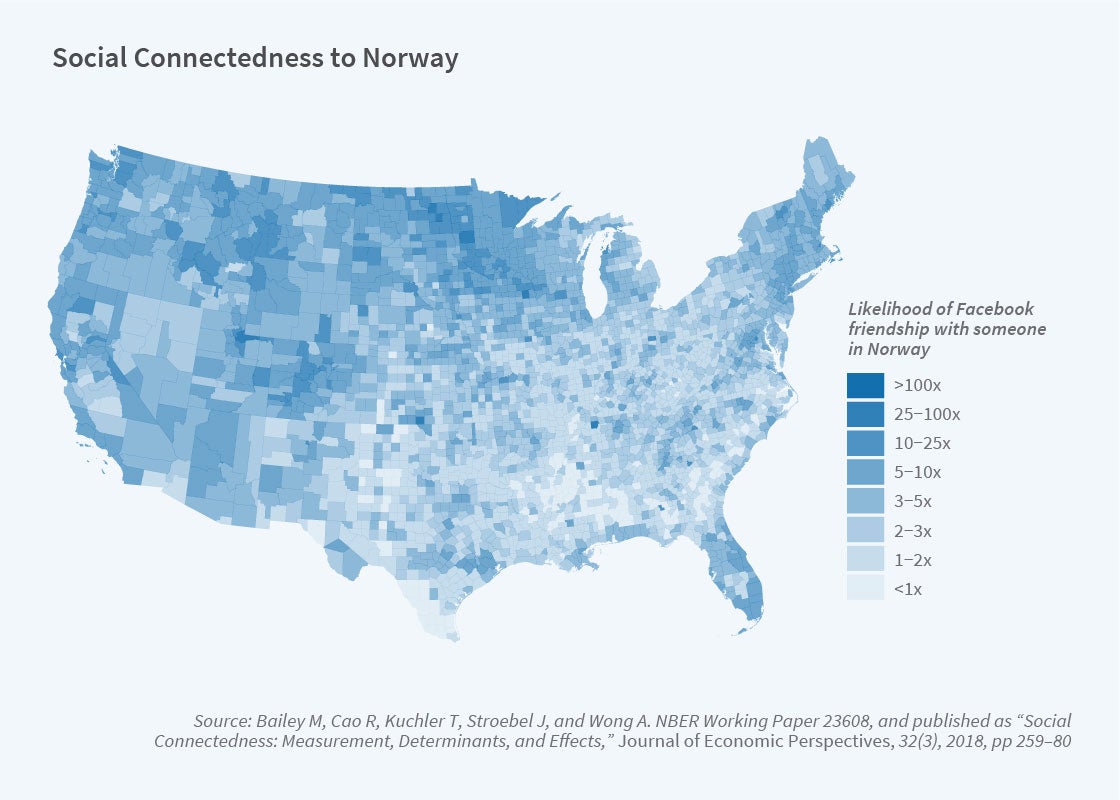
In addition to the just-described examples, the SCI is available at various levels of geographic aggregation and reveals interesting patterns in the determinants and effects of social connectedness. We explore this in a number of papers.
Together with Bailey and Patrick Farrell, we explore the structure of urban social networks within New York City and find that social connectedness is strongly determined by the structure of public transit networks.7
With Bailey, Johnston, Russel, and Bogdan State, we study the structure of social networks across European regions and find that social connectedness declines strongly at country borders and increases in migration flows.8 Importantly, we also find that historical borders and unions — such as those of the Austro-Hungarian Empire, Czechoslovakia, and eastern and western Germany — shape present-day social connectedness over and above today’s political boundaries and other controls, highlighting again the importance of historical forces in determining present-day social connectedness.
Social Connectedness and Economic Outcomes
In addition to exploring determinants of social connections, we also worked with the SCI data across a number of projects to understand the effects of these social connections on a wide range of outcome variables. In the original paper introducing this index, we showed that social connectedness strongly correlates with economic outcomes such as migration and patent citations, as well as cross-state trade flows in the United States. In more recent work with Russel, we documented that the SCI data can help improve forecasting models for infectious diseases such as COVID-19.9
In work with Yan Li, Lin Peng, and Dexin Zhou, we studied the role of social connections in the investment decisions of professional investors.10 We first showed that these investors are more likely to invest in firms located in regions to which the investor’s location has stronger social ties. This effect of social proximity on investment behavior is distinct from the effect of geographic proximity. In fact, we find that stronger social networks between geographically closer locations explain the pervasive home bias in mutual fund investments found in earlier work. The effect of social connections is largest for firms with low market capitalization and little analyst coverage. We found no evidence that investors generate differential returns from investments in locations to which they are socially connected. These results suggest that social networks increase investments through raising investors’ awareness of firms — in particular small and informationally opaque firms — that they may not otherwise know about. It is particularly interesting to find such effects of social networks on investment choices among professional investors, not just among less sophisticated retail investors.
Importantly, we find that the response of investment decisions to social connectedness aggregates up to influence equilibrium capital market outcomes: firms in locations with stronger social ties to places with substantial institutional capital — that is, firms with high social proximity to capital — have higher institutional ownership, higher valuations, and higher liquidity. These effects of social proximity to capital on capital market outcomes are largest for small firms with little analyst coverage. Our results therefore suggest that the social structure of regions affects firms’ access to capital and contributes to geographic differences in economic outcomes.
In work with Bailey, Abhinav Gupta, Sebastian Hillenbrand, and Robert Richmond, we used the international SCI data — both across countries and across subnational regions in Europe — to explore the effect of social connectedness on international trade flows.11 We found that two countries trade more when they are more socially connected, especially for goods about which information frictions may be large. The social connections that predict trade in specific products are those between the regions where the product is produced in the exporting country and the regions where it is used in the importing country. Once we controlled for social connectedness, the estimated effects of geographic distance and country borders on trade declined substantially. These findings suggest that social connectedness can alleviate information frictions to international trade.
Since the public release of the SCI data, other researchers have used it to study the role of social interactions across a wide range of topics, including peer effects in program participation, stock market participation, bank lending, consumption spillovers, voting choices, and the adoption of flood insurance. We hope that the wide availability of the data encourages even more researchers to join the exciting study of how social interactions affect economic activity.
Endnotes
“Social Finance,” Kuchler T, Stroebel J. NBER Working Paper 27973, October 2020.
“Social Networks and Housing Markets,” Bailey M, Cao R, Kuchler T, Stroebel J. NBER Working Paper 22258, May 2016. Published as “The Economic Effects of Social Networks: Evidence from the Housing Market,” Journal of Political Economy 126(6), December 2018, pp. 2224–2276.
“House Price Beliefs and Mortgage Leverage Choice,” Bailey M, Dávila E, Kuchler T, Stroebel J. NBER Working Paper 24091, November 2017, and Review of Economic Studies 86(6), November 2019, pp. 2403–2452.
“Peer Effects in Product Adoption,” Bailey M, Johnston D, Kuchler T, Stroebel J, Wong A. NBER Working Paper 25843, May 2019.
“Social Networks Shape Beliefs and Behavior: Evidence from Social Distancing during the COVID-19 Pandemic,” Bailey M, Johnston D, Koenen M, Kuchler T, Russel D, Stroebel J. NBER Working Paper 28234, December 2020.
“Measuring Social Connectedness,” Bailey M, Cao R, Kuchler T, Stroebel J, Wong A. NBER Working Paper 23608, July 2017. Published as “Social Connectedness: Measurement, Determinants, and Effects,” Journal of Economic Perspectives 32(3), Summer 2018, pp. 259–280.
“Social Connectedness in Urban Areas,” Bailey M, Farrell P, Kuchler T, Stroebel J. NBER Working Paper 26029, July 2019, and Journal of Urban Economics 118, July 2020, article 103264.
“The Determinants of Social Connectedness in Europe,” Bailey M, Johnston D, Kuchler T, Russel D, State B, Stroebel J. Social Informatics, 12th International Conference on Social Informatics, Pisa, Italy, October 6–9, 2020, pp. 1–14.
“The Geographic Spread of COVID-19 Correlates with the Structure of Social Networks as Measured by Facebook,” Kuchler T, Russel D, Stroebel J. NBER Working Paper 26990, April 2020, and Journal of Urban Economics, forthcoming.
“Social Proximity to Capital: Implications for Investors and Firms,” Kuchler T, Li Y, Peng L, Stroebel J, Zhou D. NBER Working Paper 27299, June 2020.
“International Trade and Social Connectedness,” Bailey M, Gupta A, Hillenbrand S, Kuchler T, Richmond R, Stroebel J. NBER Working Paper 26960, April 2020, and Journal of International Economics, forthcoming.