Program Report: Development of the American Economy
The Development of the American Economy (DAE) program was one of the first research programs launched by Martin Feldstein in 1978 when he formalized the modern structure of the NBER.
The mission of the program is to research historical aspects of the American economy. Its members are economic historians whose specific interests span many subfields within economics, including macroeconomics, labor economics, finance, political economy, trade, and industrial organization. Broadly, economic history research comes in two flavors. First, economic historians study the evolution of economic trends that illuminate issues relevant to the modern economy, such as the entry of women in the labor force and the moderation of economic crises over time. Second, economic historians use the natural experiments offered by history to test economic theory and identify causal channels that may drive important economic change.
Recent work by Robert Margo demonstrates the continued integration of economic historians into mainstream economics.1 Of articles published in the field’s flagship journals such as The Journal of Economic History and Explorations in Economic History, 20 percent contained econometric language in 1970—words like “regression”—and now 70 percent do. Earlier cohorts of economic historians published 36 percent of their articles in economics journals (outside of economic history journals), whereas more recent cohorts publish 75 percent of their articles in these venues.
Given the wide span of interests and expertise of DAE program affiliates, research topics cover many areas, including health, the environment, banks, financial crises, corporate governance, education, migration and immigration, and intergenerational mobility. In recent years, affiliates have responded to global events by increasing their focus on historical pandemics, industrial policy, and trade. Text analysis, the use of large language models, and machine learning are among the new methodological areas of interest used in the group.
This report highlights research in four areas: innovation and manufacturing, economic mobility, banking and finance, and women in the economy.
Innovation and Manufacturing
The creation and diffusion of innovative modes of production are central to the process of modern economic growth. These technological changes often take decades to unfold. Economic historians are, therefore, especially well positioned to gather data on these processes and interpret the evidence. DAE affiliates have investigated and illuminated the connections between science, innovation, productivity, and policy.
One strand of this research studies important changes in American technology and productivity. A canonical example of technological change and diffusion entailed the replacement of waterpower by steam power in manufacturing establishments. By digitizing and analyzing federal Census of Manufactures manuscripts from 1850 to 1880, Hornbeck, Hsu, Humlum, and Rotemberg document that as the costs of steam power declined, areas without ready access to waterpower experienced relatively fast growth in manufacturing activity.2 Moreover, using a dynamic model of firm entry and steam adoption, they show that the high fixed costs of switching to steam caused a socially inefficient lag in the technology’s diffusion, as some firms remained locked into the older, lower fixed-cost waterpower technology.
The productivity gains associated with factory mechanization were unevenly shared. Atack, Margo, and Rhode show that the rise of mechanized factories with increased division of labor led to the de-skilling of manufacturing employment in the late nineteenth century; that is, skilled craftspeople were replaced by less-skilled operatives and laborers.3 Decades later, this trend toward mechanization entailed the widespread adoption of computerized machine tools. Boustan, Choi, and Clingingsmith find that industries highly exposed to computerization experienced relatively rapid productivity growth along with reduced employment of production workers.4 Workers initially employed in these industries tended to shift into other less-impacted lines of manufacturing.
Federal policy plays a role in influencing the size and composition of the manufacturing sector, but tracing this influence requires detailed data and a long-term perspective. Klein and Meissner study tariffs and US manufacturing from 1870 to 1909 using highly disaggregated information.5 They conclude that industries with higher tariffs had lower labor productivity, higher output prices, and higher employment, and that “tariffs are unlikely to have helped the US become a globally competitive manufacturer” in this era.
In the twentieth century, World War II and the Cold War ushered in an era of expansive federal funding for R&D and innovation. Gross and Sampat examine the long-term effects of the Committee on Medical Research established during World War II on postwar biomedical innovation, the pharmaceutical industry, and National Institutes of Health research funding.6 In related work, Azoulay, Gross, and Sampat review the history of “indirect costs” in government-funded research at universities over the last 80 years.7 Kantor and Whalley show how the Cold War’s space race spurred R&D spending by NASA contractors, which increased manufacturing value added and employment in space-related sectors, albeit with modest effects on broad-based productivity.8 Giorcelli shows how US managerial practices spread to Europe, in part through the US government’s Technical Assistance and Productivity Program which allowed European managers to get training at US firms in the 1950s, and contributed to narrowing international productivity gaps.9
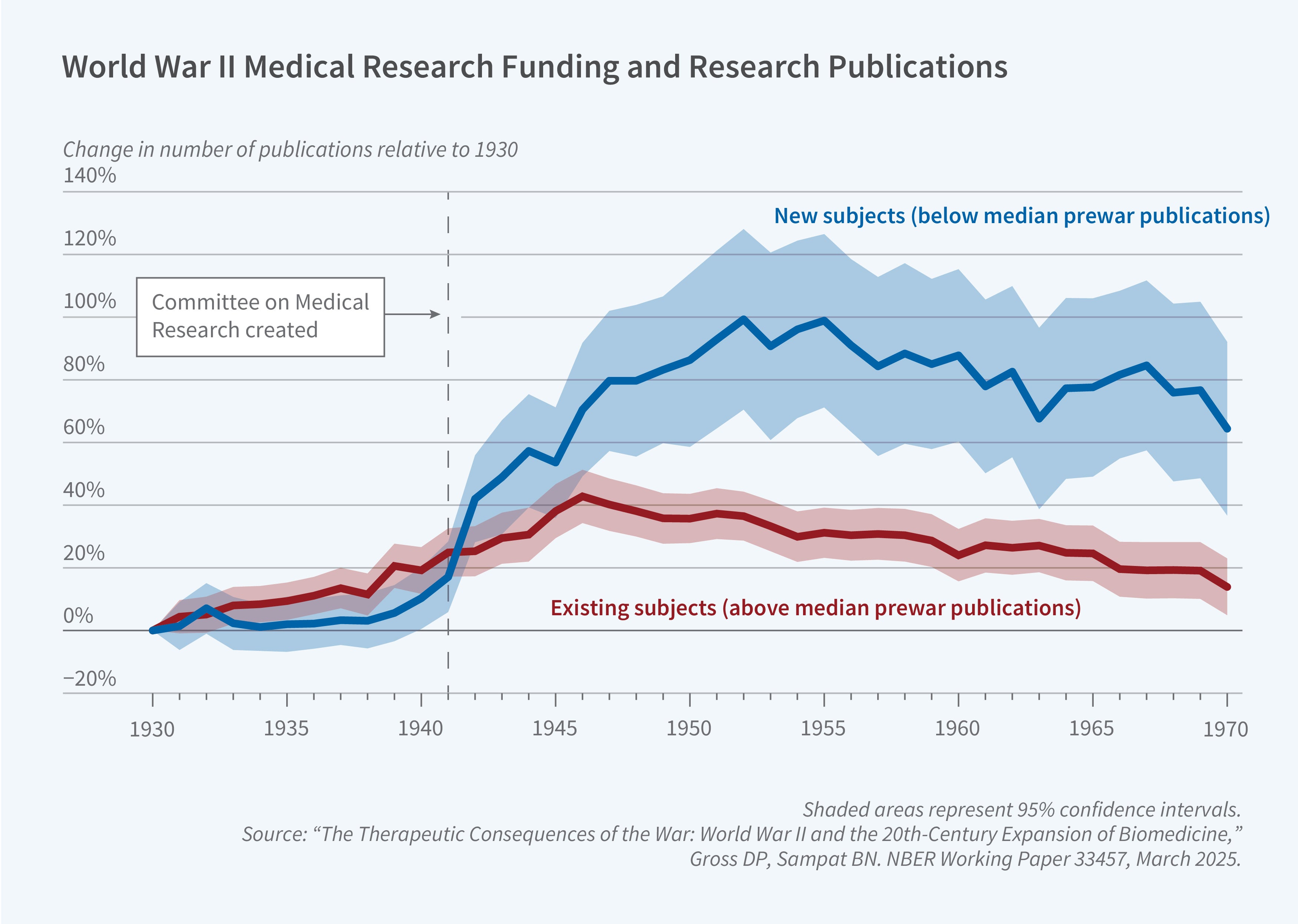
Beyond new ideas, sustained technological progress requires refinement, application, widespread adoption, and eventually adaptation to new settings. For most of human history, sustained positive rates of technological improvement were elusive. That changed during Britain’s Industrial Revolution, beginning in the eighteenth century. Hanlon sheds light on “the rise of the engineer” during the Industrial Revolution, a new kind of professional who altered the way innovations were made.10 He then incorporates this idea into a model that features a shift from “premodern” to “modern” economic growth. Rosenberger, Hanlon, and Hallmann also show how networks of innovation in Britain were important to the patterns and pace of its technological change and growth in the Industrial Revolution.11
Economic Mobility
A common view of American history holds that the US offered widespread opportunities for economic advancement, particularly in the nineteenth century when the frontier was still open to settlement and many immigrants arrived from Europe to find their fortunes, but that economic mobility has fallen since the mid-twentieth century. Economic mobility in the US has been the topic of careful empirical study in recent years, with this work summarized by Abramitzky, Boustan, and Matiashvili.12 Measuring and evaluating trends in intergenerational mobility over time is now possible due to advances in the data digitization of the complete-count US census and in historical record linkage.13
Recent research finds that intergenerational mobility was much lower in the nineteenth century than previously assumed, implying that, if anything, mobility was rising, not falling, over key decades of US history. Ward documents the importance of regional income adjustments and corrections for measurement error in obtaining accurate estimates of intergenerational mobility over time.14 Moreover, findings based on samples of White men tend to overstate intergenerational mobility in the past. New work, such as Jácome, Kuziemko, and Naidu, Collins and Wanamaker, and Buckles, Price, Ward, and Wilbert expands analysis samples to include Black men or Black and White women.15
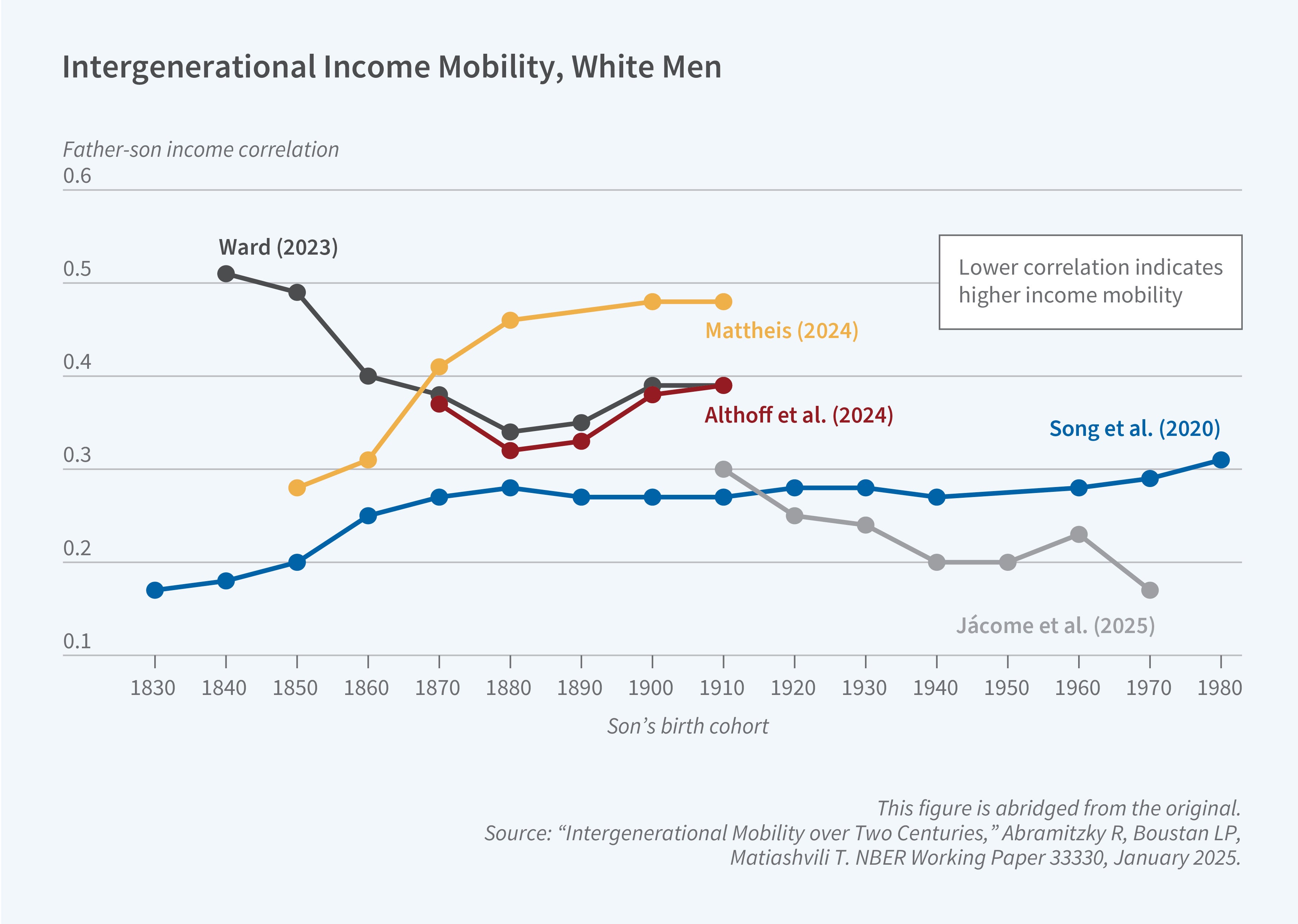
A recurrent theme is the role of military service in promoting mobility and social change in the twentieth century. Ang and Chinoy study Black veterans after World War I and illustrate how the discriminatory treatment that Black soldiers experienced in the military encouraged them to join the NAACP and become community leaders to advocate for civil rights after the war.16 A generation later, men who served in World War II enjoyed higher levels of educational attainment and enhanced income by 1950 according to Collins and Zimran, although these advantages may have dissipated by 1970.17 Barrera, Ferrara, Fishback, and Heggeness, and Abramitzky, Kowalski, Pérez, and Price document that the GI Bill helped cohorts of men from low socioeconomic status backgrounds gain access to elite colleges at mid-century, but these gains dissipated by the 1980s.18
The World War II era reshaped economic mobility beyond the direct effect on men who served in the military. Garin and Rothbaum show that regions that received federal investment for manufacturing capacity during the War experienced sustained economic growth and economic mobility in the subsequent decades.19 Collins and Niemesh analyze the homeownership boom between 1940 and 1960 and document that it was fueled both by rising real income in the postwar economy and by Veterans Administration loans.20
Immigrants and their children move up the economic ladder at a different pace than native-born Americans. Abramitzky, Boustan, Jácome, and Pérez document that the children of immigrants experienced more rapid upward mobility than the children of the US-born raised at the same point in the income distribution, both in the past and today.21 Residence in enclave neighborhoods is one important difference between immigrants and the US-born. Abramitzky, Boustan, and Connor and Abramitzky, Boustan, and Giuntella study Jewish and Polish Catholic immigrants respectively, finding that living in immigrant enclaves delayed economic assimilation while strengthening ethnic communities.22 Gagliarducci and Tabellini document a similar pattern for Italian immigrants.23
Racial gaps remain high in the US on a variety of economic metrics, including income, wealth, and intergenerational mobility. Derenoncourt, Kim, Kuhn, and Schularick provide the first continuous series of White-to-Black per capita wealth ratios from 1860 to 2020.24 This ratio is still six-to-one today, more than 150 years after Emancipation. Much of the convergence in this gap took place in the generation following the Civil War. The gap remains today in large part because of the vastly unequal initial conditions after slavery. After the Civil War, the government promised land and federal oversight of the southern labor market in the form of the Freedmen’s Bureau. Few of these promises were kept. Collins, Holtkamp, and Wanamaker examine how more widespread land ownership would have improved intergenerational mobility among Black families in the late nineteenth century.25 Chyn, Haggag, and Stuart show that southern counties that experienced more intensive federal efforts during Reconstruction experienced a backlash among White citizens measured through voting patterns and heightened racial violence.26 As a result, Black Americans had few opportunities for upward mobility in the South. Baran, Chyn, and Stuart find that moving to northern cities provided new educational opportunities for the children of Black migrants.27
Banking and Finance
Recent research has continued to advance our understanding of bank failures and financial crises. Frydman and Xu review the last 20 years of research on banking crises, highlighting the value of long-term perspective, the importance of leverage in the financial system as a precursor to crises, the negative and often long-lasting impacts on the broader economy, and the important role that timely government responses can play in offsetting panics.28
A substantial body of research examines banking during the Great Depression, when waves of bank failures fueled the economy’s downward spiral. Most places in the US lacked branch banking in the early twentieth century, a feature of the American economy that may have left it especially vulnerable to financial crises. Quincy shows that Bank of America’s branching system in California, the largest in the country, improved local credit and economic outcomes during the Depression and beyond. Bank of America’s geographic diversification and internal movement of capital insulated its branches from local shocks, in contrast to local unit banks and small branch networks.29 Mitchener and Richardson assemble new datasets from bank balance sheets and show that banks that survived the early 1930s significantly contracted their lending, accounting for a large fraction of the total decline in bank lending.30 Mitchener and Vossmeyer find that the closure of thousands of banks during the Depression did little to reduce risk in the financial system; that is, there was no “cleansing effect” from the closures.31 Instead, acquisitions merely redistributed the risk to healthier banks.
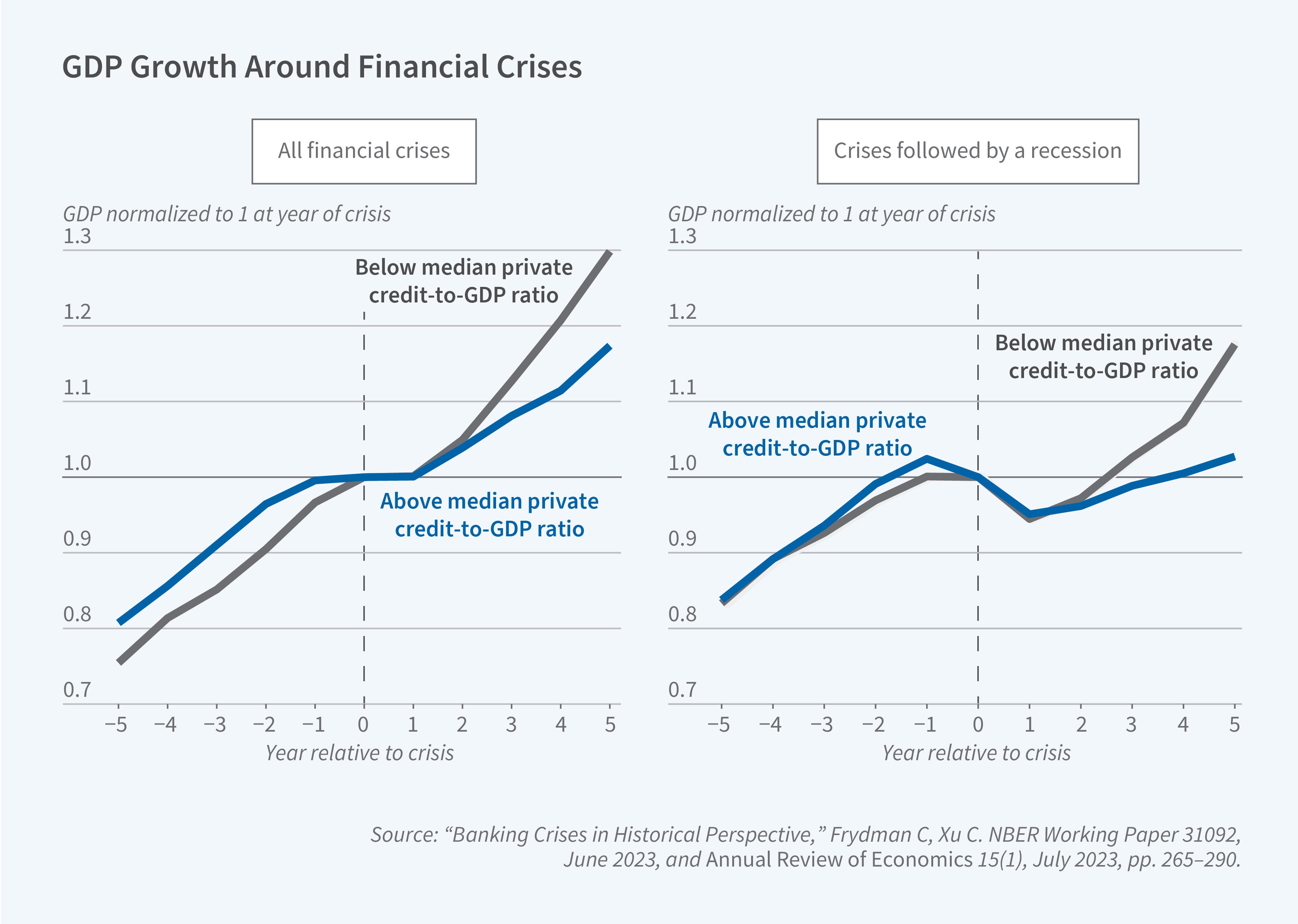
In the absence of deposit insurance, banking panics in the early years of the Great Depression were common. President Roosevelt declared a “bank holiday” in March 1933 to assess banks’ health and then allowed individual banks to re-open over time. Jaremski, Richardson, and Vossmeyer show that this sequential re-opening conveyed noisy information about banks’ health and resulted in financial resources shifting across banks and communities.32 The stigma of banks re-opening late lasted for a decade, but this was not detrimental to local commercial or industrial activity. The US Postal Savings System did insure deposits. This was a haven for depositors, but it may have exacerbated liquidity risk for local banks. Jaremski and Schuster show that banks operating near post offices that accepted deposits were more likely to close in the early years of the Depression, a reflection of depositor withdrawals.33
Recent research has also drawn on historical experience to better understand financial markets. For instance, Bernstein, Frydman, and Hilt study the introduction of Moody’s ratings for corporate securities in 1909.34 Even though the ratings had no regulatory implications, lower-than-expected ratings caused a rise in market bond yields and bonds that were rated by Moody had reduced bid-ask spreads, consistent with improved liquidity and information transmission. Cortes, Vossmeyer, and Weidenmier investigate the “war volatility puzzle” (i.e., the relatively low level of US stock volatility during wartime, even during World War II).35 They hypothesize that government-guaranteed contracts reduce the uncertainty of firms’ earnings, which is consistent with new, hand-collected military spending data spanning more than 100 years, as well as with micro-level analyses. In another paper connected to the economic ramifications of war, Brunet, Hilt, and Jaremski find that Liberty Bond drives during World War I had lasting effects on households’ financial behavior, as evidenced in mid-twentieth-century data on stock and bond ownership.36
Women in the Economy
The 2023 Nobel Memorial Prize in Economic Sciences was awarded to Claudia Goldin, who served as director of the DAE program for nearly three decades, for her research on the evolution of women’s labor force participation over the past two centuries. Goldin’s work shows that, in the early twentieth century, women often left the labor force upon marriage. By the 1960s, women instead began planning for careers, delaying marriage and childbearing to invest in higher education. Goldin considers the roles of changing social norms and legislation to formally protect women’s economic rights.37 Ngai, Olivetti, and Petrongolo construct a data series of hours spent on market work for men and women over 150 years.38 Unlike men, whose work hours have steadily declined since 1880, women’s hours first declined and then rose with the transition from agriculture and manufacturing to services and the marketization of household tasks.
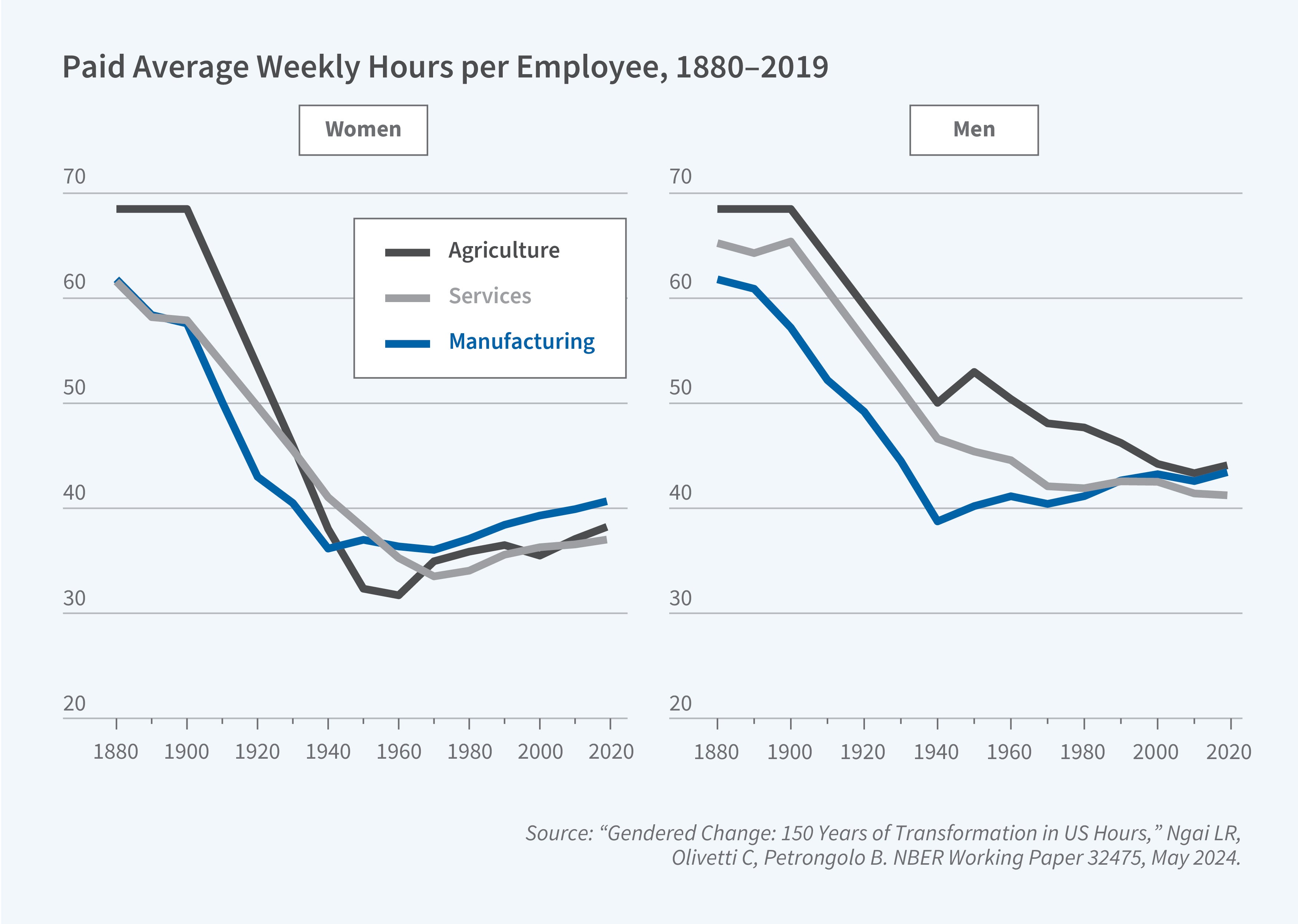
In addition to long-run economic trends, women’s fertility and work decisions have been influenced by historical events and specific policy changes. Dettling and Kearney argue that the establishment of modern fixed-rate mortgages during the Great Depression and the diffusion of these loans through the Federal Housing Administration and the Veterans Administration contributed to rising fertility during the postwar years and the baby boom.39 Goldin adds that the baby boom was an anomaly during a century otherwise marked by fertility decline as the opportunity cost of childbearing rose alongside women’s economic opportunities.40 Rising housing prices, as documented by Lyons, Shertzer, Gray, and Agorastos, may also play a role in recent fertility declines.41 Bailey, Helgerman, and Stuart document that two pieces of landmark legislation—the Equal Pay Act of 1963 and Title VII of the Civil Rights Act of 1964—contributed to the falling gender pay gap, particularly in industries more exposed to these new legal requirements, even if compliance with the law was slow.42
The reorganization of economic activity during wartime also affected women’s labor force participation. Aneja, Farina, and Xu demonstrate that the hiring of women for civil service positions during World War I had long-run effects on shaping gender norms.43 Men who worked in offices with new female coworkers raised daughters who themselves were more likely to work. Ferrie, Goldin, and Olivetti study the first government-funded childcare centers designed to allow mothers to enter the workforce during World War II.44 They find that due to limited scope and program delays, childcare did not contribute much to labor force entry.
Women have participated in entrepreneurial activities and ownership throughout US history. Wishart and Logan document that White women were actively involved in the market for enslaved persons.45 In the New Orleans market, for example, women were listed as the owner of record for 30 percent of transactions. Gozen, Hornbeck, Humlum, and Rotemberg study female-owned manufacturing establishments in the mid-nineteenth century.46 These firms were smaller than male-owned firms, employed more women, and were concentrated in subindustries like women’s clothing and millinery. Later, in the mid-twentieth century, female-owned employment agencies advertised more skilled occupations for women and helped place women workers into higher paying jobs, according to Hunt and Moehling.47
Endnotes
“The Integration of Economic History into Economics,” Margo RA. Cliometrica 12, January 2018, pp. 377–406.
“Gaining Steam: Incumbent Lock-in and Entrant Leapfrogging,” Hornbeck R, Hsu SHM, Humlum A, Rotemberg M. NBER Working Paper 32384, April 2024.
“De-skilling: Evidence from Late Nineteenth Century American Manufacturing,” Atack J, Margo RA, Rhode P. NBER Working Paper 31334, June 2023, and Explorations in Economic History 91, January 2024, Article 101554.
“Computerized Machine Tools and the Transformation of US Manufacturing,” Boustan LP, Choi J, Clingingsmith D. NBER Working Paper 30400, June 2024.
“Did Tariffs Make American Manufacturing Great? New Evidence from the Gilded Age,” Klein A, Meissner CM. NBER Working Paper 33100, February 2025.
“The Therapeutic Consequences of the War: World War II and the 20th-Century Expansion of Biomedicine,” Gross DP, Sampat BN. NBER Working Paper 33457, March 2025.
“Indirect Cost Recovery in US Innovation Policy: History, Evidence, and Avenues for Reform,” Azoulay P, Gross DP, Sampat BN. NBER Working Paper 33627, March 2025.
“Moonshot: Public R&D and Growth,” Kantor S, Whalley AT. NBER Working Paper 31471, March 2024.
“Closing the Productivity Gap with the US: Causes and Consequences of the Productivity Program in Western Europe,” Giorcelli M. NBER Working Paper 31959, January 2024.
“The Rise of the Engineer: Inventing the Professional Inventor During the Industrial Revolution,” Hanlon WW. NBER Working Paper 29751, February 2022.
“Innovation Networks in the Industrial Revolution,” Rosenberger L, Hanlon WW, Hallmann C. NBER Working Paper 32875, August 2024.
“Intergenerational Mobility over Two Centuries,” Abramitzky R, Boustan LP, Matiashvili T. NBER Working Paper 33330, January 2025. The studies referenced in figure 2 are “Intergenerational Mobility in American History: Accounting for Race and Measurement Error,” Ward Z. American Economic Review 113(12), December 2023, pp. 3213–3248. “Spurious Mobility in Imperfectly Linked Data Trials,” Mattheis R. Harvard University Working Paper. “The Missing Link(s): Women and Intergenerational Mobility,” Althoff L, Gray HB, Reichardt H. Stanford University Working Paper. “Long-Term Decline in Intergenerational Mobility in the United States since the 1850s,” Song X, Massey C, Rolf K, Ferrie J, Rothbaum F, Xie Y. PNAS 117(1), January 2020, pp. 251–258. “Mobility for All: Representative Intergenerational Mobility Estimates over the Twentieth Century,” Jácome E, Kuziemko I, Naidu S. Journal of Political Economy 133(1), January 2025.
These methods were highlighted in the NBER’s Methods Lectures series at the Summer Institute in 2017.
“Intergenerational Mobility in American History: Accounting for Race and Measurement Error,” Ward Z. NBER Working Paper 29256, January 2023, and American Economic Review 113(12), December 2023, pp. 3213–3248.
“Mobility for All: Representative Intergenerational Mobility Estimates over the 20th Century,” Jácome E, Kuziemko I, Naidu S. NBER Working Paper 29289, September 2022. “African American Intergenerational Economic Mobility Since 1880,” Collins WJ, Wanamaker MH. NBER Working Paper 23395, April 2021, and American Economic Journal: Applied Economics 14(3), July 2022, pp. 84–117. “Family Trees and Falling Apples: Historical Intergenerational Mobility Estimates for Women and Men,” Buckles K, Price J, Ward Z, Wilbert HEB. NBER Working Paper 31918, November 2024.
“Vanguard: Black Veterans and Civil Rights after World War I,” Ang D, Chinoy S. NBER Working Paper 33460, February 2025.
“World War II Service and the GI Bill: New Evidence on Selection and Veterans’ Outcomes from Linked Census Records,” Collins WJ, Zimran A. NBER Working Paper 32774, December 2024.
In 2024, with support from the Lynde and Harry Bradley Foundation, several DAE program affiliates organized a conference on The Economic Impacts of World War II. See also “The Impact of World War II Army Service on Income and Mobility in the 1960s by Ethnoracial Group,” Barrera SE, Ferrara A, Fishback PV, Heggeness ML. NBER Working Paper 33382, January 2025, and Explorations in Economic History 97, July 2025, Article 101687, and “The G.I. Bill, Standardized Testing, and Socioeconomic Origins of the US Educational Elite Over a Century,” Abramitzky R, Kowalski JK, Pérez S, Price J. NBER Working Paper 33164, November 2024.
“The Long-Run Impacts of Public Industrial Investment on Local Development and Economic Mobility: Evidence from World War II,” Garin A, Rothbaum JL. NBER Working Paper 32265, March 2024.
“Income Gains and the Geography of the US Home Ownership Boom, 1940 to 1960,” Collins WJ, Niemesh G. NBER Working Paper 31249, May 2024, and in The Economic History of American Inequality: New Evidence and Perspectives, Bailey MJ, Boustan LP, Collins WJ, editors, pp. 87–121. Chicago: University of Chicago Press, 2025, and forthcoming in Income Gains and the Geography of the US Home Ownership Boom, 1940 to 1960, Collins WJ, Niemesh GT, editors. Chicago: University of Chicago Press.
“Intergenerational Mobility of Immigrants in the US over Two Centuries,” Abramitzky R, Boustan LP, Jácome E, Pérez S. NBER Working Paper 26408, October 2019.
“Leaving the Enclave: Historical Evidence on Immigrant Mobility from the Industrial Removal Office,” Abramitzky R, Boustan LP, Connor D. NBER Working Paper 27372, June 2023. “Enclaves and Assimilation in the Age of Mass Migration: Evidence from Ethnic Catholic Churches,” Abramitzky R, Boustan LP, Giuntella O. NBER Working Paper 33362, January 2025.
“Faith and Assimilation: Italian Immigrants in the US,” Gagliarducci S, Tabellini M. NBER Working Paper 30003, April 2022.
“Wealth of Two Nations: The US Racial Wealth Gap, 1860–2020,” Derenoncourt E, Kim CH, Kuhn M, Schularick M. NBER Working Paper 30101, June 2022, and The Quarterly Journal of Economics 139(2), May 2024, pp. 693–750.
“Black Americans’ Landholdings and Economic Mobility after Emancipation: New Evidence on the Significance of 40 Acres,” Collins WJ, Holtkamp NC, Wanamaker MH. NBER Working Paper 29858, March 2022.
“Inequality and Racial Backlash: Evidence from the Reconstruction Era and the Freedmen’s Bureau,” Chyn E, Haggag K, Stuart BA. NBER Working Paper 32314, April 2024.
“The Great Migration and Educational Opportunity,” Baran C, Chyn E, Stuart BA. NBER Working Paper 31012, March 2023, and American Economic Journal: Applied Economics 16(3), July 2024, pp. 354–398.
“Banking Crises in Historical Perspective,” Frydman C, Xu C. NBER Working Paper 31092, June 2023, and Annual Review of Financial Economics 15(1), July 2023, pp. 265–290.
“Loans for the ‘Little Fellow’: Credit, Crisis, and Recovery in the Great Depression,” Quincy S. NBER Working Paper 31779, June 2024.
“Bank Lending and Deposit Crunches during the Great Depression,” Mitchener KJ, Richardson G. NBER Working Paper 32783, August 2024.
“How Do Financial Crises Redistribute Risk?” Mitchener KJ, Vossmeyer A. NBER Working Paper 31537, August 2023.
“Signals and Stigmas from Banking Interventions: Lessons from the Bank Holiday in 1933,” Jaremski MS, Richardson G, Vossmeyer A. NBER Working Paper 31088, October 2023.
“Deposit Insurance, Uninsured Depositors, and Liquidity Risk During Panics,” Jaremski MS, Schuster SS. NBER Working Paper 32284, November 2024.
“The Value of Ratings: Evidence from Their Introduction in Securities Markets,” Bernstein A, Frydman C, Hilt E. NBER Working Paper 31064, March 2023.
“Stock Volatility and the War Puzzle: The Military Demand Channel,” Cortes GS, Vossmeyer A, Weidenmier MD. NBER Working Paper 29837, May 2024.
“‘Invest!’: Liberty Bonds and Stock Ownership over the Twentieth Century,” Brunet G, Hilt E, Jaremski MS. NBER Working Paper 33541, March 2025.
“Why Women Won,” Goldin C. NBER Working Paper 31762, October 2023.
“Gendered Change: 150 Years of Transformation in US Hours,” Ngai LR, Olivetti C, Petrongolo B. NBER Working Paper 32475, May 2024.
“Did the Modern Mortgage Set the Stage for the US Baby Boom?” Dettling LJ, Kearney MS. NBER Working Paper 33446, February 2025.
“Babies and the Macroeconomy,” Goldin C. NBER Working Paper 33311, February 2025.
“The Price of Housing in the United States, 1890–2006,” Lyons RC, Shertzer A, Gray R, Agorastos DN. NBER Working Paper 32593, June 2024.
“How the 1963 Equal Pay Act and 1964 Civil Rights Act Shaped the Gender Gap in Pay,” Bailey MJ, Helgerman TE, Stuart BA. NBER Working Paper 31332, December 2023, and The Quarterly Journal of Economics 139(3), August 2024, pp. 1827–1878.
“Beyond the War: Public Service and the Transmission of Gender Norms,” Aneja A, Farina S, Xu G. NBER Working Paper 32639, June 2024.
“Mobilizing the Manpower of Mothers: Childcare Under the Lanham Act during WWII,” Ferrie JP, Goldin C, Olivetti C. NBER Working Paper 32755, January 2025.
“Her Property Transactions: White Women and the Frequency of Female Ownership in the Antebellum Era,” Wishart B, Logan TD. NBER Working Paper 32529, May 2024.
“Historical Differences in Female-Owned Manufacturing Establishments: The United States, 1850–1880,” Gozen R, Hornbeck R, Humlum A, Rotemberg M. NBER Working Paper 32575, June 2024.
“Do Female-Owned Employment Agencies Mitigate Discrimination and Expand Opportunity for Women?” Hunt J, Moehling C. NBER Working Paper 32383, April 2024.