Making the Dynamics of Social Learning Visible
Project Outcomes Statement
We study information diffusion and belief formation, set across conditions where information quality varies. We believe this can have clear implications for designing better policy. Studying how individuals process information from different sources, reconcile conflicting signals, form and update their opinions about a product or service can help expedite outreach programs of many kinds. For instance, governments and NGOs may want to know how to ensure that important information reaches potential beneficiaries.
Our first aim was to provide a low-cost methodology to infer network structure, key individuals in the network and a metric by which to measure its efficacy, allowing policy makers to efficiently use and evaluate network-based approaches.
Secondly, we aimed to examine, both theoretically and empirically, how individuals in a social network form beliefs and aggregate information.
Finally, we want to inform policy makers on how they can design efficient and cost-effective information campaigns by using the insights gained by our studies.
In our publication “Using Aggregated Relational Data to Feasibly Identify Network Structure without Network Data” we developed and tested a low-cost methodology to infer network structure and a metric by which to measure its efficacy.
The method works very well for the two empirical applications where in the past we had collected more detailed network data. At only 20% of the cost, we show that if we had used our ARD method, we would have had the exact same conclusions as in our prior papers where we had collected full network data.
This will allow a wider audience of researchers and policy makers to use and evaluate network-based approaches.
Further, we tested the common assumption in network theory that agents have full information of their network with real world network data and learned that the assumption does not hold. Allowing for incomplete network knowledge may have theoretical implications for a range of qualitative and quantitative results in various contexts.
Our results are published in “Seeing the Forest for the Trees? An Investigation of Network Knowledge”.
In our second theory paper “Naive Learning with Uninformed Agents” we studied which individuals (seeds) to select for spreading information and how their position in the network impacts the diffusion of the information. We were further interested how individuals aggregate (learn about and draw conclusions about) complex and conflicting information. Diffusion and aggregation can be thought of as occurring in two steps rather than together. First, information from seeds diffuse to all other nodes (individuals) and second, given this, aggregation starts as in the usual sense. According to the theory we developed, it is entirely possible that a large society only pays attention to a single person’s information, discarding the rest, as their diffusion influence can dominate. This can be true even in networks where everyone only has 2 or 3 links. This happens when networks are asymmetric in a very specific way. Based on the theory developed, we simulated the learning process on a set of real-world networks; these networks experience a 21.6% information loss on average. We also explored how correlation in the location of seeds can exacerbate aggregation failure. Our theory shows that in a cluster of seeds, only the information provided to the perimeter of the group affects overall social learning. The interior plays no meaningful role. Simulations with real world network data show that with clustered seeding, information loss climbs to 35%.
Our empirical work is based in rural India. This is a setting where individuals rely more on word of mouth than on media platforms to exchange information. The networks-based approach to spreading information is especially relevant in these settings. An important application of our results is to explore how to spread relevant information to a community, especially to intended beneficiaries. An allied application is to find ways to spread this information while optimizing effort and cost -- by targeting strategic individuals in a network. The network-based approach has special potential in developing country settings, where governments, NGOs and other institutions often need to reach out to a community with important information which can range from employment opportunities to health camps.
Investigators
Supported by the National Science Foundation grant #1326661
Related
Topics
Programs
More from NBER
In addition to working papers, the NBER disseminates affiliates’ latest findings through a range of free periodicals — the NBER Reporter, the NBER Digest, the Bulletin on Retirement and Disability, the Bulletin on Health, and the Bulletin on Entrepreneurship — as well as online conference reports, video lectures, and interviews.
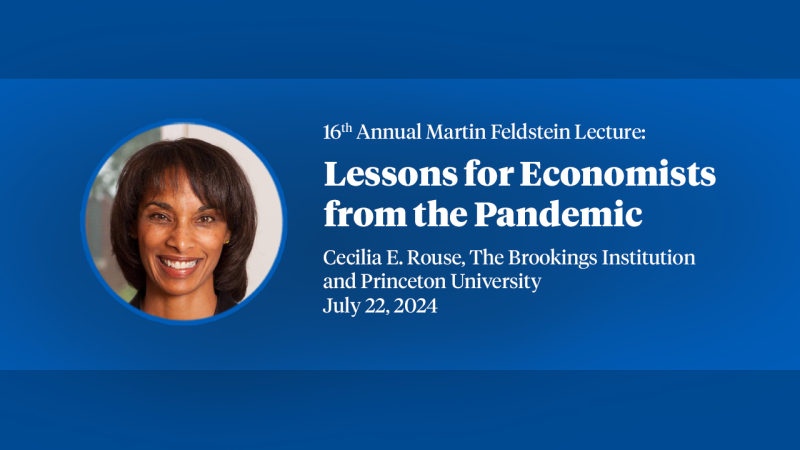
- Feldstein Lecture
- Presenter: Cecilia E. Rouse
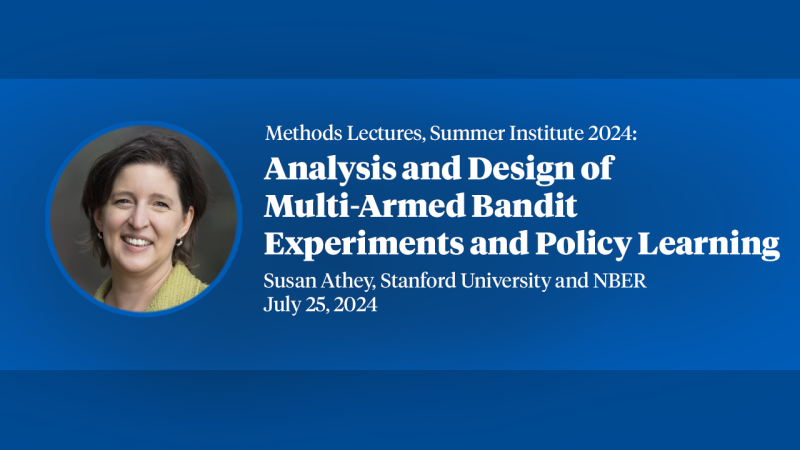
- Methods Lectures
- Presenter: Susan Athey